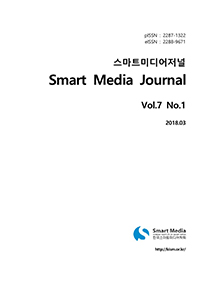
머신러닝 적용 과일 수확시기 예측시스템 설계 및 구현
(Design and Implementation of Fruit harvest time Predicting System based on Machine Learning)
- 한국스마트미디어학회
- 스마트미디어저널
- Vol8, No.1
- : KCI등재후보
- 2019.02
- 74 - 81 (8 pages)
최근에 머신 러닝 기술은 의료, 제조, 마케팅, 금융, 방송, 농업 등 사회 전반에 많은 영향을 미치고 있고 미래에도 인류의 생활에 많은 도움을 줄 것으로 예상된다. 본 논문에서는 인류의 생존에 가장 큰 영향을 주는 먹거리 즉, 농업 분야에 머신러닝 기술을 적용하는 방법을 연구한다. 농업 분야에 IoT(Internet of Things) 기술을 접목하는 스마트 팜 (Smart Farm) 분야는 생육환경을 실시간으로 모니터링 하여 농작물의 생육환경을 최적으로 유지 하는 방법을 중점적으로 연구한다. 최근 KT에서 출시된 기가 스마트 팜 솔루션 2.0 에서는 머신러닝 기술을 사용하여 온실내의 온습도를 최적으로 유지하는 기술에 머신러닝을 적용하였다. 기존의 스마트 팜 분야 연구가 생육환경 조절에 중점을 두어 생산성 증대에 집중되어 있지만 본 연구에서는 과일을 최상의 품질 상태에서 수확하여 좋은 가격으로 출하할 수 있도록 수확시기에 머신러닝을 적용하는 방법을 연구한다. 스마트팜 분야에 머신러닝 기술을 적용하기 위해서는 풍부한 빅 데이터의 확보가 무엇보다 중요하므로 정확한 머신러닝 기술을 적용하기 위해서는 지속적으로 빅 데이터 수집이 가능해야 한다. 본 논문에서 수확시기 예측에 필요한 인자로는 온실 내에서 재배되는 과일의 색상 값과 무게 값, 내부 온습도 값을 색상센서 와 무게센서, 온습도센서를 사용하여 실시간으로 수집하여 확보한다. 본 논문에서 제안하는 FPSML은 유사 과일 재배에 반복적으로 사용할 수 있는 아키텍처를 제공하며 지속적으로 빅 데이터가 축적될수록 보다 정밀한 수확시기를 예측할 수 있다.
Recently, machine learning technology has had a significant impact on society, particularly in the medical, manufacturing, marketing, finance, broadcasting, and agricultural aspects of human lives. In this paper, we study how to apply machine learning techniques to foods, which have the greatest influence on the human survival. In the field of Smart Farm, which integrates the Internet of Things (IoT) technology into agriculture, we focus on optimizing the crop growth environment by monitoring the growth environment in real time. KT Smart Farm Solution 2.0 has adopted machine learning to optimize temperature and humidity in the greenhouse. Most existing smart farm businesses mainly focus on controlling the growth environment and improving productivity. On the other hand, in this study, we are studying how to apply machine learning with respect to harvest time so that we will be able to harvest fruits of the highest quality and ship them at an excellent cost. In order to apply machine learning techniques to the field of smart farms, it is important to acquire abundant voluminous data. Therefore, to apply accurate machine learning technology, it is necessary to continuously collect large data. Therefore, the color, value, internal temperature, and moisture of greenhouse-grown fruits are collected and secured in real time using color, weight, and temperature/humidity sensors. The proposed FPSML provides an architecture that can be used repeatedly for a similar fruit crop. It allows for a more accurate harvest time as massive data is accumulated continuously.
Ⅰ. 서 론
Ⅱ. 관련연구
Ⅲ. 머신러닝 적용 과일 수확시기 예측시스템의 분석 및 설계
Ⅵ. FPSML의 프로토타이핑 및 실행
Ⅴ. 결론