BCI에서 EEG 기반 효율적인 감정 분류를 위한 LSTM 하이퍼파라미터 최적화
LSTM Hyperparameter Optimization for an EEG-Based Efficient Emotion Classification in BCI
- 한국전자통신학회
- 한국전자통신학회 논문지
- 제14권 제6호
-
2019.121171 - 1180 (10 pages)
- 263
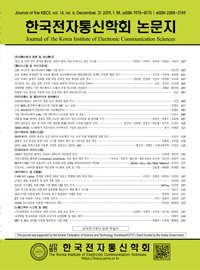
감정은 인간의 상호 작용에서 중요한 역할을 하는 심리 생리학적 과정이다. 감성 컴퓨팅은 감정을 이해하고 조절할 수 있는 인간 인지 인공 지능의 개발하는데 중점을 둔다. 우울증, 자폐증, 주의력 결핍 과잉 행동 장애 및 게임 중독과 같은 정신 질환이 감정과 관련되어 있기 때문에 이러한 분야의 연구가 중요하다. 감정 인식에 대한 노력에도 불구하고, 비정상적인 EEG 신호로부터의 감정 검출은 여전히 높은 수준의 추상화를 요구하기에 정교한 학습 알고리즘이 필요하다. 이 논문에서는 EEG 기반으로 효율적인 감정 분류를 위해 LSTM을 위한 최적의 하이퍼파라미터를 파악하고자 다양한 실험을 수행하여 이를 분석한 결과를 제시하였다.
Emotion is a psycho-physiological process that plays an important role in human interactions. Affective computing is centered on the development of human-aware artificial intelligence that can understand and regulate emotions. This field of study is also critical as mental diseases such as depression, autism, attention deficit hyperactivity disorder, and game addiction are associated with emotion. Despite the efforts in emotions recognition and emotion detection from nonstationary, detecting emotions from abnormal EEG signals requires sophisticated learning algorithms because they require a high level of abstraction. In this paper, we investigated LSTM hyperparameters for an optimal emotion EEG classification. Results of several experiments are hereby presented. From the results, optimal LSTM hyperparameter configuration was achieved.
Ⅰ. INTRODUCTION
Ⅱ. MATERIALS AND METHODS
Ⅲ. EXPERIMENT RESULTS AND PERFORMANCE ANALYSIS
IⅤ. CONCLUSION
(0)
(0)