홈보안 시스템을 위한 CNN 기반 2D와 2.5D 얼굴 인식
CNN Based 2D and 2.5D Face Recognition For Home Security System
- 한국전자통신학회
- 한국전자통신학회 논문지
- 제14권 제6호
-
2019.121207 - 1214 (8 pages)
- 137
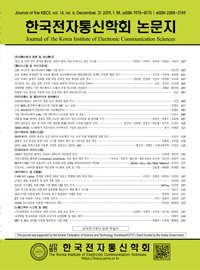
4차 산업혁명의 기술이 우리도 모르는 사이 우리의 삶 속으로 스며들고 있다. CNN이 이미지 인식 분야에서 탁월한 능력을 보여준 이후 많은 IoT 기반 홈보안 시스템은 침입자로부터 가족과 가정을 보호하며 얼굴을 인식하기 위한 좋은 생체인식 방법으로 CNN을 사용하고 있다. 본 논문에서는 2D와 2.5D 이미지에 대하여 여러 종류의 입력 이미지 크기와 필터를 가지고 있는 CNN의 구조를 연구한다. 실험 결과는 50*50 크기를 가진 2.5D 입력 이미지, 2 컨벌류션과 맥스풀링 레이어, 3*3 필터를 가진 CNN 구조가 0.966의 인식률을 보여 주었고, 1개의 입력 이미지에 대하여 가장 긴 CPU 소비시간은 0.057S로 나타났다. 홈보안 시스템은 좋은 얼굴 인식률과 짧은 연산 시간을 요구 하므로 본 논문에서 제안한 구조의 CNN은 홈보안 시스템에서 얼굴인식을 기반으로 하는 액추에이터 제어 등에 적합한 방법이 될 것이다.
Technologies of the 4th industrial revolution have been unknowingly seeping into our lives. Many IoT based home security systems are using the convolutional neural network(CNN) as good biometrics to recognize a face and protect home and family from intruders since CNN has demonstrated its excellent ability in image recognition. In this paper, three layouts of CNN for 2D and 2.5D image of small dataset with various input image size and filter size are explored. The simulation results show that the layout of CNN with 50*50 input size of 2.5D image, 2 convolution and max pooling layer, and 3*3 filter size for small dataset of 2.5D image is optimal for a home security system with recognition accuracy of 0.966. In addition, the longest CPU time consumption for one input image is 0.057S. The proposed layout of CNN for a face recognition is suitable to control the actuators in the home security system because a home security system requires good face recognition and short recognition time.
Ⅰ. Introduction
Ⅱ. Related works
Ⅲ. System configuration and the proposed methodology
Ⅳ. Simulation results and considerations
Ⅴ. Conclusions
(0)
(0)