Doc2Vec 모형에 기반한 자기소개서 분류 모형 구축 및 실험
elf Introduction Essay Classification Using Doc2Vec for Efficient Job Matching
- 한국IT서비스학회
- 한국IT서비스학회지
- 한국IT서비스학회지 제19권 제1호
-
2020.03103 - 112 (10 pages)
- 235
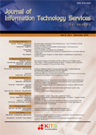
Job seekers are making various efforts to find a good company and companies attempt to recruit good people. Job search activities through self-introduction essay are nowadays one of the most active processes. Companies spend time and cost to reviewing all of the numerous self-introduction essays of job seekers. Job seekers are also worried about the possibility of acceptance of their self-introduction essays by companies. This research builds a classification model and conducted an experiments to classify self-introduction essays into pass or fail using deep learning and decision tree techniques. Real world data were classified using stratified sampling to alleviate the data imbalance problem between passed self-introduction essays and failed essays. Documents were embedded using Doc2Vec method developed from existing Word2Vec, and they were classified using logistic regression analysis. The decision tree model was chosen as a benchmark model, and K-fold cross-validation was conducted for the performance evaluation. As a result of several experiments, the area under curve (AUC) value of PV-DM results better than that of other models of Doc2Vec, i.e., PV-DBOW and Concatenate. Furthmore PV-DM classifies passed essays as well as failed essays, while PV_DBOW can not classify passed essays even though it classifies well failed essays. In addition, the classification performance of the logistic regression model embedded using the PV-DM model is better than the decision tree-based classification model. The implication of the experimental results is that company can reduce the cost of recruiting good d job seekers. In addition, our suggested model can help job candidates for pre-evaluating their self-introduction essays.
1. 서 론
2. 이론적 배경
3. 연구방법
4. 연구결과
5. 결론 및 시사점
참고문헌
(0)
(0)