Performance Comparison of Sentiment Lexicons in Predicting American Citizen s Sentiment for the Apartment Rents
- 한국지적학회
- 한국지적학회지
- 한국지적학회지 제36권 제1호
-
2020.0415 - 32 (18 pages)
- 60
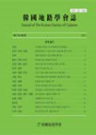
본 연구의 주된 목적은 아파트임대료에 대한 시민들의 감성을 예측하는데 있어서 어느 감성사전의 성능이 우수한지를 식별하는 것이다. 이러한 연구목적을 달성하기 위해 본 연구는 감성사전기반의 감성분석과 기계학습알고리즘기반의 감성분석을 동시에 실시하였다. 분석의 결과 AFINN Sentiment Lexicon이 모든 성과지표 범주에서 가장 성능이 우수한 감성사전으로 증명되었다. 또한 감성사전별로 아파트임대료에 대한 시민들의 감성을 분류하고 예측하는데 있어서 각 기계학습알고리즘의 성능도 뚜렷한 차이를 보였다. 즉 AFINN Sentiment Lexicon에서는 AdaBoost가, Opinion Lexicon과 NRC Emotion Lexicon에서는 Random Forest가 각각 성능이 가장 우수한 알고리즘으로 확인되었다. 본 연구는 아파트임대료에 대한 시민들의 감성을 분류하고 예측하는데 있어서 감성사전기반의 감성분석과 기계학습알고리즘기반의 감성분석을 동시에 이용했다는 점에서 이 분야의 연구에 기여할 수 있다. 이러한 장점에도 불구하고 본 연구는 제한된 범위의 감성사전과 기계학습알고리즘 만을 이용했다는 점에서 한계를 지니고 있다. 따라서 향후 연구에서는 아파트임대료에 대한 시민들의 감성을 보다 더 정확하게 분류하고 예측하기 위해 보다 많은 숫자의 감성사전과 기계학습알고리즘을 이용해야만할 것이다.
The primary purpose of this study is to identify which sentiment lexicon performs better in predicting citizens sentiment about apartment rents. To accomplish this research purpose, I performed sentiment analysis based on sentiment lexicon and sentiment analysis based on the machine learning algorithm simultaneously. As a result of the analysis, the AFINN Sentiment Lexicon turned out to be the best performing sentiment lexicon in all performance metrics categories. Also, the performance of each machine learning classifier showed a significant difference in classifying and predicting citizens sentiment on apartment rents by sentiment lexicon. In other words, AdaBoost in the AFINN Sentiment Lexicon and the Opinion Lexicon and Random Forest in the NRC Emotion Lexicon proved to be the best algorithms. This study can contribute to the research of this area because it uses sentiment analysis based on the sentiment lexicon and sentiment analysis based on the machine learning algorithm simultaneously in classifying and predicting citizens sentiment about apartment rents. Despite these advantages, this research has limitations in that it uses only a limited range of sentiment lexicons and machine learning algorithms. Therefore, future studies will have to use more sentiment lexicons and machine learning algorithms to classify and predict citizens sentiment about apartment rents accurately.
1. 서 론
2. Literature Review
3. Research Methodology
4. Research Results and Discussion
5. Conclusion
References
(0)
(0)