베이지안 시공간 모형을 이용한 한반도 오존농도 예측
Bayesian Spatio-Temporal Models for Ozone Concentration Level in South Korea
- 한국자료분석학회
- Journal of The Korean Data Analysis Society (JKDAS)
- Vol.22 No.3
-
2020.061067 - 1079 (13 pages)
-
DOI : 10.37727/jkdas.2020.22.3.1067
- 88
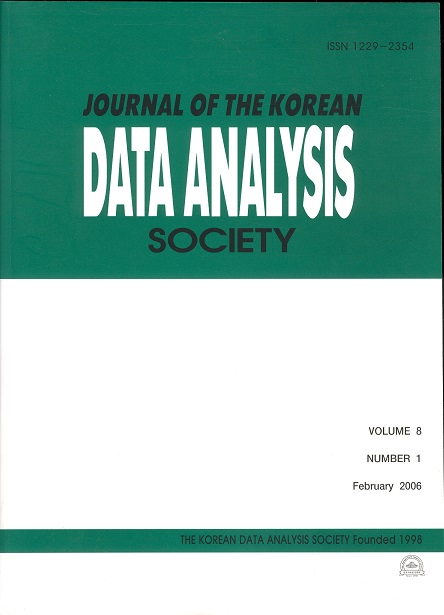
한반도의 대기오염 상태는 계절과 지역에 따라 다르게 나타나기 때문에 한반도 오존농도 분포를 시공간적 분포로 나타낼 수 있다. 본 연구에서는 한반도 오존농도를 계층적 베이지안 시공간모형을 이용하여 예측하고 시각화 하였다. 베이지안 시공간모형의 확률과정으로 독립가우시안 모형(GP model)과 자기상관모형(AR model)을 이용하였다. 연구에 사용된 자료는 우리나라 대기오염 관측소와 기상관측소에서 관측된 2014년부터 2016년까지 3년간 오존농도자료와 기상요인자료이다. 기상요인은 최대기온, 평균풍속, 상대습도, 일사량을 사용하였다. 대기오염 관측소의 위치와 기상관측소의 위치가 상이하여 기상관측소로부터 5km이내에 있는 33개의 대기오염 관측소만 이용하여 분석을 수행하였다. 베이지안 시공간 모형에서는 공간상관성을 설명하는 모수인 Φ의 사전분포를 설정하는 것이 중요하다. 그러나 Φ의 사후분포가 수렴하지 않아 한반도 오존농도의 RMSE가 가장 낮은 최적의 Φ값을 찾았다. Geweke 진단법을 이용하여 베이지안 모형의 MCMC 수렴도를 평가하였고 RMSE, MAE, MAPE, BIAS를 이용하여 GP모형과 AR모형을 비교분석 하였다. 마지막으로 97개소 ASOS 기상데이터를 이용하여 울릉도, 제주도, 백령도 등 대기오염 관측소가 없는 지역까지 한반도의 전역의 오존농도를 시각화 하였다.
Environmental has a property of space-time. Having both the spatial and temporal dimensions makes substantial complexity to environmental data analysis. In this study we built models using daily maximum 8-hour ozone concentration data obtained from 33 sites in South Korea for analysis between 2014 and 2016. Maximum temperature, relative humidity, and wind speed were considered as covariates. The data on the square root scale seems most good in terms of both stabilizing and symmetry the variance. We specified Independent Gaussian process model and Autoregressive model as the probability process of hierarchical Bayesian framework and Markov Chain Monte Carlo techniques. These spatio-temporal models allow spatial prediction of a temporally aggregated ozone summary along with its uncertainty. Although the autoregressive parameter was statistically significant, Independent Gaussian process model well reflected the spatio-temporality of ozone concentration level on the Korean Peninsula.
1. 서론
2. 연구방법론
3. 연구자료
4. 연구결과
5. 결론
References
(0)
(0)