k-중앙개체 군집방법을 이용한 한국 프로농구선수의 군집화
Clustering Korean Professional Basketball Players by Using k-Medoids Clustering
- 한국자료분석학회
- Journal of The Korean Data Analysis Society (JKDAS)
- Vol.10 No.6
-
2008.123423 - 3433 (11 pages)
- 71
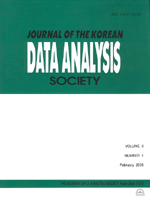
주어진 데이터의 개체들을 비슷한 특징을 가지는 소그룹으로 나누어 그 그룹들의 특징이나 대표성을 찾는 분석 과정을 군집분석이라고 한다. 군집분석은 크게 분리 군집방법과 계층적 군집방법으로 구분할 수 있다. 본 연구에서는 계층적 군집방법을 이용하여 군집의 수를 정하고, 분리 군집방법 중 하나인 k-중앙개체 군집방법을 적용하여 한국 프로농구선수들의 군집화를 시도해 보았다. 프로농구선수들의 데이터는 몇몇 변수들에 있어 특이치가 존재하기 쉽다. 따라서 이런 경우에는 특이치에 영향을 크게 받는 k-평균 군집방법을 적용하는 것보다는 특이치에 덜 민감한 k-중앙개체 군집방법의 활용이 좋은 결과를 줄 수 있다. k-중앙개체 군집방법의 구현을 위해 PAM(partitioning around medoids) 알고리즘을 이용하였다. 군집분석결과 3개의 군집으로 선수들을 군집화하였고, 각 군집의 특징을 파악하였다.
Cluster analysis is one of statistical methods for finding groups so that objects in the same group are similar each other and objects in the different group are dissimilar. There are two distinctive techniques in cluster analysis. One is hierarchical method the other is partitioning method. In this study, we built the clusters from the data of korean professional basketball players. The hierarchical method was used for finding the proper number of clusters and k-medoids clustering which is one of partitioning method was used for building clusters. The professional basketball players data generally has outliers in several variables. Therefore, instead of applying k-means clustering for this kind of data, k-medoids clustering which is not affected a lot by outliers can give a better result than that of k-means clustering. In order to implement k-medoids clustering PAM(partitioning around medoids) algorithm was used. The resulting clusters are obtained as three distinguished clusters and the characteristics of each cluster are summarized.
1. 서론
2. 분리 군집방법
3. 군집결과
4. 결론
참고문헌
(0)
(0)