Applications to Bayesian Model Selection Problems via Stochastic Approximation Monte Carlo
- 한국자료분석학회
- Journal of The Korean Data Analysis Society (JKDAS)
- Vol.10 No.6
-
2008.123043 - 3056 (14 pages)
- 3
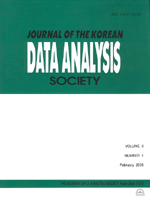
Stochastic approximation Monte Carlo algorithm has recently been proposed by Liang, Liu and Carroll(2007) as a general simulation and optimization algorithm. In this paper, we discuss the application of this algorithm to Bayesian model selection problems and propose the new sampling method for the case of the multiple change-points identification among those problems. We estimate multiple change-points in the Bayesian multiple change-points model when the data are from normal distributions. The algorithm is tested on simulated and the real dataset, and compared with evolutionary Monte Carlo algorithm. The numerical results indicate that the new sampling method can generate the efficient samplers and thus Stochastic approximation Monte Carlo outperforms evolutionary Monte Carlo significantly for the model selection problems.
1. Introduction
2. Stochastic approximation Monte Carlo
3. Bayesian Model Selection Problems
4. Numerical results
5. Conclusion
Reference
(0)
(0)