로지스틱 회귀를 이용한 의사결정 나무의 분기점 선택에 대한 연구
A Study on Decision Tree using Logistic Regression Coefficients
- 한국자료분석학회
- Journal of The Korean Data Analysis Society (JKDAS)
- Vol.10 No.3
-
2008.061517 - 1526 (10 pages)
- 14
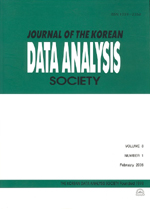
많은 의사결정 나무 방법은 분리변수(split variable) 선택에 있어 범주형 분리변수에 대해 편향을 가지고 있다. 즉 많은 범주를 갖는 범주형 변수가 분리 변수로 더 많이 선택되는 경향이 있다. 이에 Loh & Shih(1997)는 변수선택에 있어서 무시할만한 편향을 가지며 통계적 검정법을 사용하는 QUEST(Quick, Unbiased, Efficient, Statistical Tree) 방법을 제안하였다. 그러나 이 방법은 분류 정확도와 최종나무크기 면에서는 우세하다고 할 수 없다.본 논문에서는 범주형 변수에 대한 편향의 문제를 해결하는 방법으로, 범주형 변수를 독립변수로 한 로지스틱 회귀모형을 구축하여 각 범주에 해당하는 로지스틱 회귀계수를 이용해 범주들을 순위로 변환한 후 지니 지수(Gini index)를 의사결정나무 분석에 사용하는 분류나무를 제안하였다. 또한 실제 데이터를 이용하여 모형의 정확도(accuracy)와 최종나무크기(tree size) 측면에서 기존의 방법과 비교하였다.
There are some selection bias of splitting categorial variables. Variables which have more categories tend to be selected as split variables. Loh and Shih (1997) have proposed a statistical tree QUEST(Quick, Unbiased, Efficient, Statistical Tree) which is not biased to select split variables.In this study, we propose a decision tree method using logistic regression coefficient as a transformation method which transforms categorical variables to ordinal. Then we apply CART to transformed data. Finally we compare the proposed method with previous decision tree applying real data sets in the view of tree sizes and accuracies.
1. 서론
2. 의사결정나무
3. 로지스틱 회귀계수를 이용한 의사결정나무
4. 사례분석
5. 결론
참고문헌
(0)
(0)