불균형 데이터에 대한 오버샘플링 효과 연구
The Effect of Oversampling Method for Imbalanced Data
- 한국자료분석학회
- Journal of The Korean Data Analysis Society (JKDAS)
- Vol.10 No.4
-
2008.082089 - 2098 (10 pages)
- 42
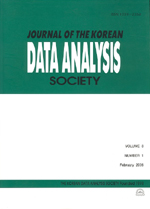
대용량 데이터로부터 의미있는 지식을 발견하는 마이닝 과정에서 모형구축을 위한 표본추출은 필수적이라 할 수 있다. 그러나 목표변수가 단지 두 가지의 결과만을 가지고 있고 관심 범주의 사례 수가 드물게 발생하는 경우가 있다. 이와 같이 전체 모집단에서의 목표변수의 관심 사례 집단의 비율이 매우 적은 경우에는 일반적으로 단순임의추출법이 아닌 오버샘플링 방법이 자료분석가들에게 추천되고 있다. 본 연구에서는 이러한 오버샘플링의 활용 지침을 위하여 사례집단의 비율에 따른 오버샘플링 효과를 분석하였으며 모의실험 결과는 다음과 같았다. 즉, 모집단에서의 사례집단과 대조집단의 불균형 비율이 1:5를 넘어서는 경우 오버샘플링 방법을 사용하는 것이 효과적이고 오버샘플링의 비율은 1:2와 1:3의 사이에서 가장 효과적인 결과를 도출 할 수 있는 것으로 연구되었다.
In data mining process, it is necessary for prediction modelling to sample data from the large dataset. However, when the particular events of interest are quite rare in the dataset, their consequences can be quite dramatic and quite often in a negative sense. That is, standard data mining schemes do not work well for rare class analysis. In this case, oversampling method is recommanded by many dataminers to solve these problems. In this paper, we study the effects of oversampling method and suggest the good rule of oversampling rate. Simulation results show that the optimal oversampling rate ranges from 1:2 to 1:3. This results will be helpful for the dataminers.
1. 서론
2. 오버샘플링
3. 데이터 및 연구설계
4. 결론
참고문헌
(0)
(0)