다변량 의생명 자료분석을 위한 자기조직도의 활용 연구
Applications of Self-Organizing Map for Multivariate Biomedical Data
- 한국자료분석학회
- Journal of The Korean Data Analysis Society (JKDAS)
- Vol.10 No.4
-
2008.081887 - 1901 (15 pages)
- 3
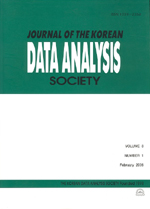
다변량 의생명 자료를 분석하기 위한 군집분석으로 계층적 군집분석이나 k-평균 군집분석, 자기조직도, 주성분 분석 등이 많이 사용되고 있다. 이 중에서 자기조직도(self-organizing map; SOM)는 군집에 대한 정보를 시각화된 그래프로 제공한다는 장점이 있으며, 패턴인식에 유용한 것으로 연구된 바 있다. 이 연구에서는 자기조직도의 기본 알고리즘과 성질을 정리하고, 자기조직도의 여러 변종들에 대해 조사하였다. 또한 결측치 처리방법이나 여러 사전 조건에 따라 결과가 어떻게 달라지는지 실제 마이크로어레이 데이터분석과 모의실험을 통하여 알아보았다. 그 결과 사전조건의 변화에 따라 군집결과는 달라지며, 그리드의 형태나 중량의 초기치 선정방법에 따른 변화보다는 국소가중치함수의 선정이나 학습률의 함수형태에 더 영향을 받음을 알 수 있었다. 결측치 대체방법으로는 평균이나 중앙값을 사용한 것보다 KNN을 사용한 것이 원 데이터의 결과와 보다 가까운 결과를 가져왔다.
To analyze the multivariate biomedical data, many clustering methods such as hierarchical clustering, k-means clustering, self-organizing map and principal component analysis are used. Among them, the self-organizing map (SOM) is known to have merit to provide visualized information and useful for pattern recognition. In this study, we summarize the basic algorithms and properties of SOM and investigate several variants. We compare the performance of the results according to missing imputation methods and several different predefined parameters by analyzing real microarray data and simulation data. The clustering results are more sensitive to weight function or learning rate function than grid type or initial weights.
1. 서론
2. 자기조직도의 알고리즘과 분석요인
3. 자기조직도의 변종
4. 분석사례
5. 모의실험
6. 결론
참고문헌
(0)
(0)