공통요인 분석자 혼합모형의 요인점수를 이용한 로지스틱 신용분류
Credit Classification using Logistic Model with Factor Scores of Mixtures of Common Factor Analyzers
- 한국자료분석학회
- Journal of The Korean Data Analysis Society (JKDAS)
- Vol.10 No.5
-
2008.102605 - 2613 (9 pages)
- 22
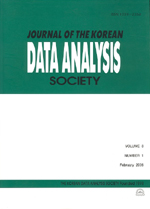
로지스틱판별분석은 금융 분야의 계량분석에서 유용하게 사용되고 있는 통계적기법이다. 또한, 신용평점자료를 이용하여 신용평가를 함에 있어서도 로지스틱판별분석은 해석이 쉽고 우수한 판별력으로 많이 활용되고 있다. 하지만 연속형설명변수의 수가 대단히 많은 경우 적절한 모형 선택을 해야하는 어려움이 발생한다. 이에 대한 대안으로서 다수의 연속형설명변수들을 공통요인분석자혼합모형에 의한 차원축소를 통해 새로운 소수의 특징변수로 변환할 수 있다. 본 연구에서는 공통요인분석자혼합모형에 의하여 변환된 소수의 요인점수들을 로지스틱판별분석의 새로운 연속형설명변수로 사용하여 신용상태를 분류하는 방법을 제시한다. 실제 자료인 호주의 신용평점자료를 이용하여 일반적인 로지스틱판별분석과 새로운 소수의 요인점수를 이용한 로지스틱판별분석과의 정분류율의 차이를 비교하였다. 실험결과 공통요인분석자혼합모형을 이용하여 구한 새로운 요인점수를 이용한 로지스틱판별분석의 분류 성능이 더 우수하였다.
Logistic discrimination is an useful statistical technique for quantitative analysis of financial service industry. Especially for the credit classification using credit scoring data, it is not only easy to be implemented, but also has good classification rate. However in case where there are many explanatory variables in the logistic model, we need model selection procedure to identify significant variables for the model. We propose a dimension reduction method instead of model selection, generating small number of factor scores by mixtures of common factor analyzers(MCFA), and use them as new features in the model. In this study we propose to use factor scores of MCFA as new feature variables in the logistic model for credit classification. We used a real credit scoring data to compare the performance between the classical logistic model with model selection and the proposed method. It was shown that the proposed method using low dimensional factor scores outperformed the conventional logistic model.
1. 서론
2. 공통요인 분석자 혼합모형을 이용한 차원축소
3. 실제 금융회사의 신용평가 자료를 이용한 로지스틱판별분석
4. 결론 및 토의
참고문헌
(0)
(0)