연관성 규칙에서의 향상도 기반 흥미도 측도의 제안
The Development of Lift-based Interestingness Measure in Association Rule Mining
- 한국자료분석학회
- Journal of The Korean Data Analysis Society (JKDAS)
- Vol.10 No.5
-
2008.102595 - 2603 (9 pages)
- 12
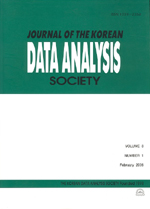
데이터마이닝은 방대한 양의 데이터 속에서 쉽게 드러나지 않는 유용한 정보를 찾아내는 기법으로서 각종 데이터를 기반으로 감춰진 지식, 기대하지 못했던 경향 또는 새로운 룰 등을 발견하는데 사용된다. 데이터마이닝 기법으로는 연관성규칙, 군집 분석, 의사결정나무기법, 신경망모형, 자기조직화지도 등의 분석 기법이 있다. 이들 중에서 연관성규칙은 데이터베이스 내에 존재하는 항목들 간의 상호 관련성을 찾아내는 기법으로서 항목들 사이의 지지도, 신뢰도, 향상도 등의 흥미도 측도를 기준으로 상호 관련성 여부를 측정한다. 본 논문에서는 향상도가 가지고 있는 방향성과 범위의 제한이 없는 단점을 보완하는 동시에 흥미도 측도가 가져야 할 조건들을 만족하는 새로운 흥미도 측도를 제안하고자 한다.
Data mining is the method to find useful information for large amounts of data in database. One of the well-studied problems in data mining is exploration for association rules. An association rule technique finds the relation among each items in massive volume database. Some interestingess measures have been developed in association rule mining. Interestingness measures are useful in that it shows the causes for pruning uninteresting rules statistically or logically. This paper present a new lift-based interestingess measure(we call pure lift) to evaluate association rules and describe some properties for a proposed measure. A comparative study with some interestingess measures is shown by numerical example. The results show that the pure lift can avoid the discovery of misleading association rules.
1. 서론
2. 순수 향상도의 제안
3. 에제를 통한 고찰
4. 결론
참고문헌
(0)
(0)