연관성 규칙 발견을 위한 군집분석의 적용 방안
A Study of k-means Clustering for Association Rule
- 한국자료분석학회
- Journal of The Korean Data Analysis Society (JKDAS)
- Vol.9 No.6
-
2007.122919 - 2930 (12 pages)
- 9
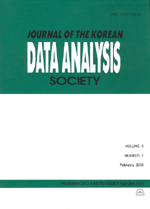
연관성 규칙은 대용량 데이터베이스에서 각 항목들 간의 관련성을 찾아내는 기법으로, 연관성 규칙 생성 시 지지도와 신뢰도 및 향상도의 값을 연구자가 임의로 지정하여 규칙을 생성한다. 이 때 어느 한 값이라도 주어진 조건에 맞지 않으면 규칙이 생성되지 않는다. 예를 들어 지지도와 향상도 값은 매우 높으나 신뢰도 값이 지정된 값보다 조금 작을 경우를 생각해 보면, 이 규칙은 의미 있는 규칙이라 볼 수 있으나 기존의 연관성 규칙에서는 주어진 조건에 맞지 않아서 규칙이 생성되지 않으므로 의미 있는 규칙을 발견할 수 없다. 결과적으로 우리가 모르는 사이에 의미 있는 규칙이 누락되는 오류를 범하게 된다. 이에 본 논문에서 연관성 규칙에 의해 생성된 규칙을 군집화 하여 기존 방법의 연관성 규칙에서는 발견할 수 없는 의미 있는 규칙을 발견하고자 한다.
An association rule technique finds the relation among each items in massive volume database. In generating association rules, the researcher specifies the measurements randomly such as support, confidence and lift, and produces the rules. The rule is not produced if it is not suitable to the one any condition which is given value. For example, in case of a little small than the value which a confidence value is specified but a support and lift s value is very high, this rule is meaningful rule. But association rule mining can not produce the meaningful rules in this case because it is not suitable to the condition which is given value. Consequently, we creat insignificant error which is not selected to the meaningful rules. In this paper, we suggest clustering to association rule measures for finding effective association rules.
1. 서론
2. 이론적 배경
3. 연관성 규칙 발견을 위한 군집분석의 적용 방안
4. 적용 사례
5. 결론
참고문헌
(0)
(0)