Variable Selection Algorithm in Classification Tree
- 한국자료분석학회
- Journal of The Korean Data Analysis Society (JKDAS)
- Vol.3 No.4
-
2001.12361 - 369 (9 pages)
- 7
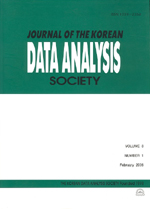
A classification tree is a set of rules to classify and to predict the class of a target variable from one or more predictor variables. Main steps for constructing a tree consist of data partitioning, tree simplification, and model fitting. We especially focus on the topic of variable selection which is a very important step in constructing classification trees. In this paper we suggest an improved variable selection algorithm based on Kruskal-Wallis test which is a nonparametric competitor of F -test used in QUEST. We show through a Monte Carlo simulation that the proposed algorithm has some desirable properties in terms of bias and power when selecting a predictor variable.
1. Introduction
2. Variable Selection Algorithms
3. Kruskal-Wallis Test Based Variable Selection
4. Conclusion
References
(0)
(0)