A Study on Regression Tree for Count Data with a Data mining Application on Industrial Accidents
- 한국자료분석학회
- Journal of The Korean Data Analysis Society (JKDAS)
- Vol.5 No.2
-
2003.06137 - 144 (8 pages)
- 이용수 2
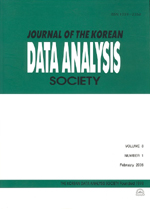
키워드
초록
Decision tree as one of many data mining techniques is a popular approach for segmentation, classification and prediction by applying a series of simple rules. In general, to analyze continuous target variable, we use F-statistics or variance reduction criterion to find the best split. But these methods are only appropriate to a continuous target variable. If the target variable is discrete, especially count data, above criteria couldn’t give a good result to analyst because of its attribute. In this paper, we will propose a decision tree for count data, rare event, using maximum poisson likelihood as split criterion and using Korean industrial accident data sets, we will compare the performance of the split criteria.
목차
1. Introduction
2. Homogeneity measure using ML
3 Application
4 Conclusions and Discussion
References
참고문헌 (0)
등록된 참고문헌 정보가 없습니다.
해당 권호 수록 논문 (0)
등록된 수록 논문 정보가 없습니다.