정규혼합모형의 대용량자료 적합을 위한 일반화 Incremental EM 알고리즘에 대한 연구
A Generalized Increment EM algorithm for fitting Normal Mixture Model in Large Data Set
- 한국자료분석학회
- Journal of The Korean Data Analysis Society (JKDAS)
- Vol.6 No.4
-
2004.081031 - 1042 (12 pages)
- 8
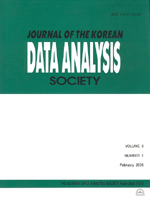
IEM(Incremental EM) 알고리즘은 자료의 개수가 매우 클 때 정규혼합모형을 추정하기 위해 자주 사용된다. 이 알고리즘은 자료를 여러 개의 블록으로 분할한 후 각 집단에 대해 부분 E-단계를 처리하는 기법으로서, 자료의 크기 때문에 발생하는 EM의 비실현성 문제를 해결할 뿐만 아니라 알고리즘 자체의 수렴속도를 향상시킨다. 그러나 많은 응용문제에서 자료 블록이 고정되어 있고 또 블록의 개수가 매우 큰 경우가 자주 나타난다. 이 경우 IEM 알고리즘 역시 실현성의 문제점이 발생한다. 이 문제를 해결하기 위해 본 연구에서는 블록 집합 크기의 구성을 통해 EM알고리즘 뿐 아니라 IEM 알고리즘을 포함하는 보다 일반화된 알고리즘을 제안한다. r차-IEM알고리즘이라 부르는 이 알고리즘을 통해 블록의 개수가 매우 클 때 최대 로그-우도값에 도달하는 처리시간이 EM이나 IEM 알고리즘보다 더 짧은 차수 r이 존재함을 모의실험을 통해 보였다.
The IEM(Incremental Expectation Maximization) algorithm is frequently used to fit normal mixture model when data sets are large. For the algorithm, dataset underlying is divided into several blocks available and the partial E-step performs for each block. However, this method still suffers inefficiency of cpu time for the case in which the numbers of blocks are fixed and large. In this paper, an algorithm so called the rth-ordered IEM algorithm is proposed which provides a general form in a sense that it constructs a class of IEM algorithms with the size of block sets. With the rth-ordered IEM, when the size of block set are large we find that there exists the order(say r) such that it has a less arrival time to maximum of the log-likelihood than those of the EM and the IEM algorithm through a simulation study.
1. 서론
2. IEM 알고리즘과 문제제기
3. 제안된 IEM 알고리즘
4. 모의실험
5. 결론
참고문헌
(0)
(0)