Robust Least Squares Support Vector Machine
- 한국자료분석학회
- Journal of The Korean Data Analysis Society (JKDAS)
- Vol.6 No.4
-
2004.08943 - 951 (9 pages)
- 2
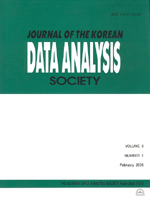
The least squares support vector machine(LS-SVM) is a widely applicable and useful machine learning technique for classification and regression analysis. LS-SVM is an SVM(support vector machine) version which involves equality constraints instead of inequality constraints and works with a squared loss function, which leads the solution to be obtained from a linear Karush-Kuhn-Tucker conditions instead of a quadratic programming problem. A drawback of LS-SVM is that the estimates is less robust due to the assumption of the errors and the use of a squared loss function. In this paper we propose a robust LS-SVM which imposes the robustness on the estimation of LS-SVM by eliminating the candidates of outliers. The proposed method are also applied to pruning support vectors in the LS-SVM case. In the numerical studies, the performance of the robust LS-SVM is shown and compared with the ordinary LS-SVM via FVU.
1. Introduction
2. Robust LS-SVM Regression
3. Robust Pruning Procedure
4. Numerical Study
5. Concluding Remarks
References
(0)
(0)