A Bayesian Method for Gene Selection in the Weibull Survival Model with Application to DNA Microarray Data
- 한국자료분석학회
- Journal of The Korean Data Analysis Society (JKDAS)
- Vol.6 No.4
-
2004.08917 - 928 (12 pages)
- 2
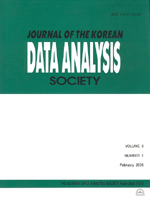
In this paper we consider the well-known Weibull regression models for survival analysis. These models are usually used with few covariates and many observations (subjects). But, for a typical setting of gene expression data from DNA microarray, we need to consider the case where the number of covariates p exceeds the number of samples n . For a given vector of response values which are times to event(death or censored times) and p gene expressions(covariates), we address the issue of how to reduce the dimension by selecting the significant genes. This approach enables us to estimate the survival curve when n≪p . In our approach, rather than fixing the number of selected genes, we will assign a prior distribution to this number. The approach creates additional flexibility by allowing the imposition of constraints, such as bounding the dimension via a prior, which in effect works as a penalty. To implement our methodology, we use a Markov Chain Monte Carlo(MCMC) method. We demonstrate the use of the methodology to diffuse large B-cell lymphoma(DLBCL) complementary DNA(cDNA) data and Breast Carcinomas data.
1. Introduction
2. Weibull Regression Model for Gene Selection
3. Examples
4. Discussion
5. Apendix
References
(0)
(0)