인자분석자 혼합모형을 위한 Incremental EM 알고리즘
Incremental EM algorithm for Normal Mixtures of Factor Analyzer
- 한국자료분석학회
- Journal of The Korean Data Analysis Society (JKDAS)
- Vol.7 No.5
-
2005.101605 - 1614 (10 pages)
- 5
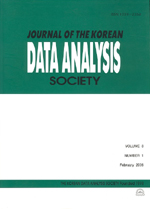
최근 차원축소된 고차원 특질변수를 사용하는 정규혼합모형의 적합을 위해 인자분석자 정규혼합모형이 자주 사용된다. 그런데 많은 응용문제에서 자료들이 한 곳에 저장되어있기 보다는 여러 서버에 분할 저장되어있는 경우가 많다. 이런 분할자료들을 한 곳에 모으기는 현실적으로 어려울 뿐 아니라, 설혹 가능하다 하더라도 병합된 자료가 너무 대용량이어서 모형적합을 위해 EM 알고리즘을 사용이 거의 불가능할 경우가 있다. 이런 경우 병합된 자료를 일괄 처리하기보다는 분할된 자료집단을 연속적으로 방문하면서 처리하는 방법을 고려하거나 병합된 자료를 몇 개의 블록으로 분할하여 처리하는 것이 현실적이다. 이러 경우 incremental 타입의 알고리즘이 필요하다. 이에 본 논문에서는 인자분석자 정규혼합모형의 적합을 위해 사용되는 AECM 알고리즘의 incremental EM 타입을 제시하며, 그 타당성과 수렴성질을 조사한다.
Nowadays the normal mixtures of factor analyzer is frequently used to fit the normal mixture model with highly dimensional feature variables by using lower-dimensional factors. In applications it is not seldom that the dataset are distributed over the severs rather than placed at one archive. And it is not a simple problem to gather them at one place because its gathered dataset is too big, and because gathering work itself is burdensome even if it is possible. In this case, rather than a batch processing, it is more practical to use a partial processing while visiting each server continuously. This paper provides an incremental EM version of the AECM algorithm for the normal mixtures of factor analyzer for a large and/or distributed dataset. And for the algorithm some validities and properties of convergence are presented.
1. 서론
2. 인자분석자 정규혼합모형
3. 모형 적합을 위한 AECM-IEM 알고리즘
4. AECM-IEM 알고리즘의 수렴성질
5. 실험
6. 결론
참고문헌
(0)
(0)