CMAQ 예측치를 이용한 서울지역 PM10 농도 예측모형
Prediction Model of PM10 Concentration over Seoul using CMAQ Forecasts
- 한국자료분석학회
- Journal of The Korean Data Analysis Society (JKDAS)
- Vol.18 No.6
-
2016.123001 - 3009 (9 pages)
- 68
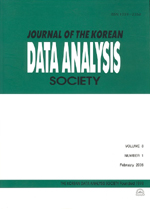
미세먼지 예보는 대기질 예측 수치모형인 CMAQ 모형(community multiscale air quality model)에서 생성된 PM10 농도예측치를 바탕으로 네 범주(좋음, 보통, 나쁨, 매우나쁨)로 발표되고 있다. 본 연구는 PM10 농도에 대한 계량치 예측의 정확도를 향상시키기 위한 역학-통계모형 개발을 목적으로 하였다. 예보대상지역은 서울이며, 3시간단위 초단기 예보시스템에 적용이 가능하도록 예측모형을 개발하고자 하였다. 계량치 예측모형을 개발한 후 생산된 농도예측치를 바탕으로 네 범주 예측치를 생산하는 과정으로 이루어진다. 모형개발에 사용된 자료는 PM10 농도에 대한 관측치와 해당 시점에 대한 CMAQ 예측치를 상용하였다. 자료기간은 3개월(2014년 3월1일 - 5월31일)이다. CMAQ 예측치는 과소예측하는 체계적 오차를 가지고 있다. 체계적 오차를 감소시키기 위한 역학-통계모형으로 동적선형모형을 적용하였다. 동적선형모형에서 생성된 PM10 농도 예측치를 네 범주예보에 적용한 결과 정확도가 향상된 것으로 나타났다. 특히 피해가 상대적으로 큰 고농도 경우 우수한 개선 결과를 보이고 있다.
Air quality forecast system announces four-categorical forecast (good, normal, bad, and very bad) based on CMAQ (community multiscale air quality model) forecasts. The present study aims to improve forecast accuracy of PM10 concentration over Seoul area using physical-statistical modeling methods. For the 3-hour forecast system, we used observations of PM10 concentration and the corresponding CMAQ forecasts for a period of three months (from Mar 1 to May 31 in 2014). According to examine systematic error of CMAQ forecasts, dynamic linear model (DLM) is applied as a physical-statistical model. The generation of forecasts consists of two steps. Firstly, quantitative forecasts can be generated using the trained DLM. Secondly, four-categorical forecasts can be determined based on DLM forecasts. As results, DLM reduces systematic error in CMAQ forecasts and accuracy of DLM forecasts are better than that of CMAQ forecasts.
1. 서론
2. 자료 및 연구방법
3. 동적선형모형 예측 결과
4. 결론
References
(0)
(0)