엔트로피 기반 연관성 평가 기준의 제안
Proposition of Entropy Based Association Thresholds
- 한국자료분석학회
- Journal of The Korean Data Analysis Society (JKDAS)
- Vol.18 No.4
-
2016.081905 - 1914 (10 pages)
- 4
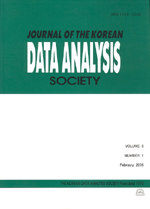
오늘날 빅 데이터 기술이 발전하고 전문적인 서비스가 다양화됨에 따라 정부뿐만 아니라 기업에서는 빅 데이터로부터 실질적인 가치를 창출하고 새로운 기회를 탐색하고 있다. 데이터 마이닝은 빅 데이터 내에 있는 유용한 정보를 발견하고 이를 바탕으로 의사결정 등을 위한 정보로 활용하고자 하는 것이다. 데이터 마이닝 분야에서 널리 이용되고 있는 연관성 규칙은 흥미도 측도를 근거로 항목들 간의 관련성 여부를 측정하며, 연관성의 방향에 따라 정의 연관성 규칙, 역의 연관성 규칙, 그리고 부의 연관성 규칙으로 나누어진다. 기본적인 연관성 평가 기준인 지지도, 신뢰도, 그리고 향상도는 정의 연관성 규칙만을 생성하기 위한 것으로 역 또는 부의 연관성 규칙을 고려하지 않음으로 인하여 의미 없는 규칙이 생성될 수도 있고 의미 있는 규칙이 생성되지 않을 수도 있다. 본 논문에서는 의사결정나무에서 활용되고 있는 엔트로피 기반의 측도들 중에서 결합 엔트로피, 조건부 엔트로피, 그리고 상호 정보량 등을 변형하여 기본적인 연관성 평가 기준을 대체할 수 있는 측도를 제안하였다. 그 결과, 기본적인 연관성 측도는 모두 양의 값만을 취하는 반면에 엔트로피 기반 측도들은 모두 양의 값뿐만 아니라 음의 값을 동시에 취하는 것으로 나타났다. 따라서 엔트로피 기반 측도들을 연관성 평가 기준으로 활용하게 되면 연관성의 방향을 파악할 수 있다는 사실을 확인하였다.
These days, government and companies are creating real value from big data and explore new opportunities by big data technology and diversified professional services. By Wikipedia, data mining is the computational process of discovering patterns in huge data sets involving methods at the intersection of artificial intelligence, machine learning, and statistics. Association rule is one of the well-established methods in data mining, and can be divided into positive, negative, and inverse rules. It discovers associations among items in a big data on the basis of several association thresholds (support, confidence, and lift). If we use these thresholds for association rule generation, we cannot know whether the association is positive or negative by their values, and so we may reach the wrong conclusion. In this paper, we proposed three kinds of entropy-based modified measures to compensate for this disadvantage, and compared some various aspects through a few experiments. The results showed that they had the direction of association.
1. 서론
2. 엔트로피 기반 연관성 평가 기준
3. 예제를 통한 고찰
4. 결론
References
(0)
(0)