연관성 규칙 관점에서 수정된 헬링거 측도의 제안
Proposition of Adjusted Hellinger Measure in Association Rule Mining
- 한국자료분석학회
- Journal of The Korean Data Analysis Society (JKDAS)
- Vol.18 No.1
-
2016.02199 - 206 (8 pages)
- 이용수 5
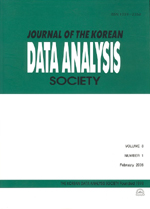
초록
위키 백과사전에 의하면 데이터 마이닝은 대규모로 저장된 데이터 안에서 체계적이고 자동적으로 통계적 규칙이나 패턴을 찾아내는 것으로 고객 관계 관리, 신용평가모형 개발, 사기탐지시스템, 장바구니 분석, 최적 포트폴리오 구축 등과 같이 다양한 산업 분야에서 광범위하게 사용되고 있다. 이들 데이터 마이닝 기법 중의 하나인 연관성 규칙은 연관성 평가 기준에 의해 관심 있는 항목들 간에 관련성을 찾아내는 기법으로 많은 연구자들에 의해 평가 기준들이 개발되어 왔다. 이들 중에서 헬링거 측도는 연관성 규칙의 관점에서 볼 때 여러 가지 연관성 측도들에 비해 많은 장점이 있으나 양의 연관성의 정도와 음의 연관성의 정도를 합해서 결과를 도출하기 때문에 연관성의 방향을 판단하기가 곤란한 문제를 내포하고 있다. 이를 해결하기 위해 본 논문에서는 헬링거 측도를 변형한 수정된 헬링거 측도를 제안하고 예제를 통하여 비교하였다. 그 결과, 연관성의 방향을 제시하는 수정된 헬링거 측도가 기존의 측도보다 더 바람직한 것으로 나타났다.
By Wikipedia, data mining is the computational process of discovering patterns in large data sets involving methods at the intersection of artificial intelligence, machine learning, statistics, and database systems. It has been utilized in many industrial sectors such as credit scoring system, fraud detection system, customer relationship management, and decision making. Association rule, one of the well-established methods in data mining, finds the relationship between antecedent items and consequent items in a huge database. Evaluation criteria of association rules have been developed by many researchers. Among them, the Hellinger measure is the association threshold considering the information content and the generality of a rule. If we use the Hellinger measure for rule generation, we cannot determine the direction of the association. In this paper we proposed a adjusted Hellinger measure to compensate for this disadvantage, and we investigated some useful properties for this measure. And then we compared some various aspects through a few experiments. The results showed that the adjusted Hellinger measure was better than the Hellinger measure because it had the right direction of association.
목차
1. 서론
2. 수정된 헬링거 측도의 제안
3. 적용 예제
4. 결론
References
참고문헌 (0)
등록된 참고문헌 정보가 없습니다.
해당 권호 수록 논문 (44)
- 남자대학생의 음주결과기대, 대인관계능력 및 우울이 문제음주에 미치는 영향
- 은행의 재무적 상황이 주택담보대출의 변화에 미치는 영향
- 중년여성의 삶의 질에 영향을 미치는 요인
- 전문계고 학생의 학교생활만족도가 취업 후 현재일자리 만족도에 미치는 영향에서 진로성숙도의 매개효과 검증
- Binary Forecast of Haze over Busan
- 협력적 필터링에서 순위일치도 향상을 위한 결측치 대체 방법
- 암환자를 돌보는 간호사의 직무 스트레스와 공감능력이 소진에 미치는 영향
- 개인의 성격속성이 위험기피도의 가상편의에 미치는 효과
- 연령별 출산율 적합을 위한 모수적 모형에 대한 연구
- 건강/수명 경로를 통한 의료비지출과 지역경제성장 관계에 대한 실증적 분석
- 대학생의 전공계열별 스트레스 인지와 대처, 대학생활 적응의 관계
- 재무곤경위험과 주식수익률
- 청소년 비만에 영향을 미치는 개인수준과 학교수준 요인의 효과
- 연관성 규칙 관점에서 수정된 헬링거 측도의 제안
- Bayesian Estimation of Exponentiated Weibull SSPALT Model using Type-I Hybrid Censoring
- 초등학교 고학년 학생의 인터넷 과다사용에 영향을 미치는 요인
- 복합 포아송 분포를 이용한 0과 양수값이 혼재된 강수자료 분석
- The Role of Credit Default Swaps in the Korean Stock Market
- 연속형과 범주형의 혼합자료에 대한 혼합형 h-plot
- The Nexus between Financial Status of Credit Card Business and Macro Economic Factors: Evidence from Korean Credit Card Firms
- Threshold Models for Count Time Series: Case Study
- 제임스-스타인 추정량을 이용한 부대 사격 명중률의 추정 방법
- 간호대학생의 대인관계, 우울이 스마트폰 사용수준에 미치는 영향
- 균형계통추출과 회귀추정법을 이용한 모평균 추정
- 타인의 정서적 피드백이 완벽주의자의 적응행동에 미치는 영향
- Authentic Experience and Consumer Responses in Ethnic Restaurants: The Expectation-Confirmation Perspective
- 메르스 역학 자료를 이용한 감염 Susceptible-Infected-Removed 모형 구축
- 쇼핑몰 특성이 고객만족 및 재방문 의도에 미치는 영향
- 노인의 구강건강관련 삶의 질에 영향을 미치는 요인
- 연관성 분석에서 표준화 향상도의 개선 방안 연구
- Association Analysis between the Type of Institutional Investors and the Financial Investment Behaviors
- 무관질문 확률화응답기법을 이용한 민감한 속성에 대한 모비율의 구간추정
- 현금흐름과 할인율에 의한 주식 수익률의 분해
- SUR을 활용한 노후대비 재무수단별 가입 결정요인
- 텍스트마이닝을 활용한 연준의 통화정책방향 의결문 분석
- 사회적 자본 및 파트너특성이 공동기술개발성과에 미치는 영향
- 통계청 생명표에 기초한 100세 이상 사망률 연장에 관한 연구
- 우리나라 중·고등학교의 교육프로그램 및 교육재정 투입 효과 검증
- 행사가격별 KOSPI200옵션 내재변동성의 정보효과
- 좌우 화살표로 구성된 복합자극에 대한 전역
- 남녀 음주자들의 음주 양가감정과 음주행동 및 문제의 관계에서 음주억제의 매개효과
- Generalized Partially Linear Single-index Model for Analysis of the Prevalence of Korean Type 2 Diabetes
- Sarmanov 분포를 이용한 이변량 포아송모형에 대한 추론
- Time-Varying Volatility Spillover between Gold Futures and Singapore Stock Markets: Implication of the Portfolio Management