이미지 픽셀 분류를 위한 동시검정법 모의실험 연구
A Simulation Study on Classification of Image Pixels using Location-Scale Tests
- 한국자료분석학회
- Journal of The Korean Data Analysis Society (JKDAS)
- Vol.18 No.2
-
2016.04697 - 705 (9 pages)
- 2
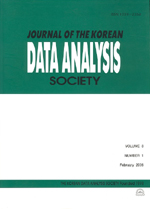
이미지 인식기술은 빅데이터 시대를 맞이하여 활발하게 활용되고 있다. 본 연구에서는 새로운 이미지(image) 픽셀(pixel) 값의 정분류율을 높일 수 있는 통계적 분류방법에 대해 제안하고자 한다. 최근 Liao, Akritas은 통계적 가설검정 기반 이미지 픽셀 분류방법을 새롭게 제안하였으나, 그들이 제안한 방법은 p-값(p-value)이 너무 작을 경우 오분류율이 높다는 단점이 있다. 이 단점을 보완하기 위해 Ghimire, Wang은 p-값이 아주 작은 경우에 평균과의 거리를 이용하여 픽셀값을 분류하는 방법을 고안하였다. 그러나 기존의 방법보다는 오분류율이 줄었지만, 집단별 분산이 다를 경우 오분류율이 높다는 단점이 있다. 따라서 본 연구에서는 집단별 분산이 다를 경우, 위치모수(location parameter)와 척도모수(scale parameter)의 동시검정법을 이용한 가설검정기반 이미지픽셀 분류방법을 새롭게 제안하고자 한다. 동시검정법으로는 Kolmogorov-Smirnov 검정, Cramér- von Mises 검정, Lepage 검정, Cucconi 검정을 고려하였고, 기존의 방법보다 두 집단의 분산이 다른 경우 제안한 방법이 보다 효율적임을 확인하였다.
Image recognition technologies are getting popular in big data ages. In this study, we suggest statistical testing procedures to improve classification rates of image pixels. Recently, Liao, Akritas (2007) suggested a classification of image pixels based on hypothesis testings which is a powerful nonparametric classification method. However, their method may misclassify many image pixels in the given image due to small p-values. So, Ghimire, Wang (2012) suggest new method for classification of image pixels using a distance to the mean in the case of very small p-values. But it also has a problem when variances of classes are different. Thus, we suggest methods for classification of image pixels based on simultaneous location-scale tests (Kolmogorov-Smirnov test, Cramér-von Mises test, Lepage test, Cucconi test). We find that location-scale tests we suggest are more efficient than previous ones when two groups are heterogeneous.
1. 서론
2. 이미지 픽셀 분류
3. 모의실험 및 결과
4. 결론 및 토의
References
(0)
(0)