분산 베이지안 추론방법과 가우시안 확률과정 분류모형을 이용한 다차원 자료의 분류
Classification of Multidimensional Data Using Variational Bayesian Gaussian Process Classification Model
- 한국자료분석학회
- Journal of The Korean Data Analysis Society (JKDAS)
- Vol.17 No.6
-
2015.123047 - 3055 (9 pages)
- 9
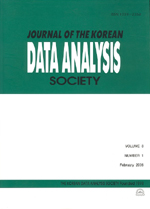
최근에 새롭게 제안되고 있는 가우시안 확률과정 분류모형에 대한 통계적 추론절차로 분산 베이지안 추론방법을 고찰하였고, 이모형을 이용하여 실제 다차원 자료를 분류할 수 있는 활용방안을 제시하였다. 먼저 우리는 다차원 자료의 분류범주를 나타내는 잠재변량에 대한 사전분포로 가우시안 확률과정을 가정하였고, 관측자료에 우도함수로 로지스틱 함수를 고려하여 잠재변량에 대한 사후분포를 유도하는데 근사적 베이지안 추론방법을 고려하였다. 이때 분류모형을 정의하기 위해서 필요한 잠재변량과 다양한 모수들에 대한 사후분포를 유도하는데 분산 베이지안 추론방법과 중요샘플기법을 사용하였고, 또한 새로운 관측 자료를 분류하는데 사용될 수 있는 잠재변량에 대한 예측분포를 유도하였다. 제안된 모형의 성능을 평가하기 위해서 두 종류의 다변량 자료를 사용하여 실험을 실시하였다. 실험결과들은 제안된 방법이 이들 자료들을 효율적으로 잘 분류함을 보여주고 있다.
In this paper, we present the variational Bayesian Gaussian process classification model for the classification of multidimensional data. Here, we have considered an efficient computational method that can be used to obtain the approximate posterior distributions for latent variables and various parameters need to define the multi-classes Gaussian process classification model. We have first induced the approximate posterior distributions for parameters and latent function by using variational Bayesian inference method and important sampling technique, and next we derived a predictive distribution of latent function used at the classification of new sample. We have conducted experiments that can verify the performance of our model for two multidimensional dataset. Experimental results show the fact that our method may classify efficiently the multidimensional data.
1. 서론
2. 가우시안 확률과정 분류모형
3. 실험결과
4. 결론
References
(0)
(0)