양적·질적 혼합형 데이터에 대한 군집분석 알고리즘 비교 및 사례분석
A Comparison and Case Study of Cluster Algorithms for Mixed Data with Quantitative and Qualitative Variables
- 한국자료분석학회
- Journal of The Korean Data Analysis Society (JKDAS)
- Vol.17 No.6
-
2015.122991 - 3002 (12 pages)
- 128
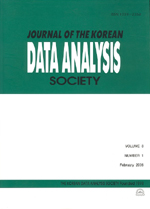
군집분석은(cluster analysis)은 군집의 개수나 구조에 관한 특별한 가정 없이 개체들 사이의 유사성 또는 거리(비유사성)에 근거하여 자연스러운 군집을 찾고 다음 단계의 분석을 꾀하는 탐색적인 통계분석 기법이다. 전통적인 거리-기반 군집분석에서는 양적(연속형) 데이터가 주로 사용된다. 그러나 질적(범주형) 데이터가 포함되어 있는 데이터에 대해서 군집분석을 수행하고자 하는 경우도 자주 발생한다. 특히 데이터마이닝과 같은 분야에서는 인구사회적 특성이나 거래 속성 등의 질적 변수를 포함하여 분석해야 하는 경우가 매우 많다. 본 연구에서는 양적·질적 변수들이 혼합되어 있는 데이터에 대하여 가장 널리 사용되는 군집분석 알고리즘들을 비교하였다. 여기에서 다루는 알고리즘은 거리에 기초한 SAS/Enterprise Miner의 k-평균 군집화, 확률분포에 기초한 SPSS의 2-단계(two-step) 군집화, 혼합모형에 기초한 Latent GOLD의 LCA(latent class analysis) 방법이다. 본 연구의 사례분석 결과에서도 볼 수 있듯이 동일한 데이터에 대하여도 사뭇 다른 결과를 산출한다. 따라서 실제 문제에서 연구자들은 한 가지 방법만으로 결과를 도출하지 말고, 다양한 방법들을 사용하여 결과를 얻고 그들을 서로 비교하여 가장 합리적인 결과를 얻을 수 있도록 노력할 필요가 있다.
Cluster analysis is an exploratory statistical method to search significant structures inherent in a given data set based on similarity or distance among observations. Conventional clustering algorithms are applied to mostly quantitative variables for which a distance can be defined naturally. However, in many application fields we are often confronted with a mixed data set which contains qualitative variables as well as continuous variables. In this study, we compare some clustering methods which are commonly used for such a mixed data by conducting simulations with real data sets. The clustering methods we consider are k-means clustering, two-step clustering and latent class analysis method which can be easily implemented using statistical softwares such as SAS/E-miner, SPSS and Latent GOLD. The comparative simulation results can be helpful to practical researchers considering a cluster analysis with a mixed numeric and categorical data set.
1. 서론
2. SAS/Enterprise Miner의 k-평균 군집분석
3. SPSS의 2-단계 군집분석
4. Latent GOLD의 LCA 방법
5. 논의 및 결론
References
(0)
(0)