지분된 범주를 가진 분류문제에 대한 계층적 분류분석 방법
A Hierarchical Approach in Nested Multicategory Classification
- 한국자료분석학회
- Journal of The Korean Data Analysis Society (JKDAS)
- Vol.11 No.1
-
2009.02339 - 347 (9 pages)
- 6
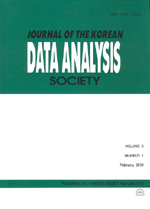
최근 gene expression 자료 또는 mass spectrometry 자료를 기반으로 하여 종양을 임상적으로 진단하는 방법들이 많은 주목을 받아왔다. 그러나 이와 같은 자료에서 정상그룹에 비해 종양을 가진 환자그룹은 종양의 진행정도에 따라 서로 다른 특징을 갖는 부집단이 존재하는 경우가 빈번하게 관찰된다. 본 논문에서는 이와 같은 하나의 특정모집단에 여러 부모집단(sub-population)이 존재하는, 지분된(nested) 자료에 대한 이단계(two-stage)의 계층적(hierarchical) 다범주 분류방법을 제안한다. 첫 번째 단계에서 정상그룹과 종양그룹을 분류하는 이범주 분류모형을 구축하고, 두 번째 단계에서는 첫 번째 단계에서 종양그룹으로 분류한 표본에 대하여, 세부 종양그룹으로 분류하는 다범주 분류모형을 구축한다. 모의실험과 실제자료 분석을 통하여 제안한 방법과 일반적인 이범주/다범주 분류방법들을 분류정확도기준으로 비교하였다.
In recent years, cancer diagnosis based on gene expression data or mass spectrometry data has received much attention. Classification of normal versus cancer patients plays an important role. In this paper, we propose a new classification procedure for classifying normal versus various types of cancers. A standard multi-category classification method can be applied to consider the normal group as an additional group. However such a naive approach has several disadvantages. First, the accuracy would be sub-optimal. Second, it would be hard to control the sensitivity and specificity. In practice, classifying normal versus any type of cancer is much more important than classifying the type of cancer for a cancer patient. To resolve these problems, we propose a two-stage classification method. In the first stage, we construct a classifier for normal versus any type of cancers. In the second stage, we construct a learner for classifying the type of cancer for those who are classified as cancer in the first stage. By analyzing simulations and real data set, we demonstrate that the proposed method is more accurate than standard multi-category approaches.
1. 서론
2. 이단계 계층적 다범주 분류방법
3. 자료분석
4. 결론 및 토의
참고문헌
(0)
(0)