다변량 혼합반응 자료를 위한 다변량 의사결정나무에 관한 연구
Multivariate Decision Tree for Mixed Responses
- 한국자료분석학회
- Journal of The Korean Data Analysis Society (JKDAS)
- Vol.11 No.2
-
2009.04851 - 858 (8 pages)
- 13
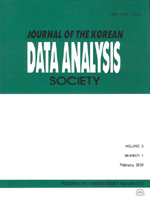
CART, CHAID, QUEST와 같이 데이터마이닝에서 자주 사용되는 대부분의 의사결정나무 알고리즘들은 반응변수가 일변량인 경우에만 분석이 가능하다. 따라서 반응변수가 다변량일 경우에는 기존의 의사결정나무분석을 수행하는데 한계가 있다. 최근 다변량 반응을 분석할 수 있는 방법으로 마할라노비스 거리를 이용한 다변량 의사결정나무, 일반화 엔트로피지수를 이용한 의사결정나무 등이 제안되어 있으나 이들은 한 가지 형태의 다변량 반응변수(연속형 변수들, 또는 범주형 변수들)에만 적용 가능하였다. 본 논문은 기존의 방법론의 계량을 통해 범주형과 연속형 변수가 혼합된 다변량 반응변수인 경우에 대한 새로운 알고리즘을 제안하고, 모의실험을 통해 마할라노비스 거리를 이용한 방법이 다소 우수함을 보였다.
In the field of data mining, decision trees can be used to classify and predict the responses of data. There are many tree methods for uni-variate responses, such as CHAID, CART and QUSET. Recently, researches based on these methods like Mahalanobis distance and Generalized Entropy Index for multivariate responses have also been studied. However, these algorithms are limited to be applied to mixed multivariate responses. In this paper, we modify previous multivariate decision trees and suggest new splitting criterions using Mahalanobis distance and Gower’s Similarity coefficient for any type of mixed responses. To compare the performances of the proposed trees, simulation studies on two new splitting criterions using Mahalanobis distance and Gower’s Similarity coefficient are performed.
1. 서론
2. 혼합반응에 대한 다변량 의사결정나무
3. 모의실험
4. 결론
참고문헌
(0)
(0)