비정규성 변수에 대하여 잠재변수를 이용한 다중대체법의 적용
Multiple Imputation Based on Latent Variables for Data Sets with Non-normal Type of Variables
- 한국자료분석학회
- Journal of The Korean Data Analysis Society (JKDAS)
- Vol.11 No.3
-
2009.061377 - 1387 (11 pages)
- 23
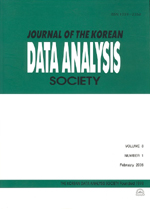
모수적 방법을 사용하여 대체(imputation)를 실시할 때 다변량 정규분포를 가정하는 경우가 흔하다. 실제 자료는 정규분포를 따르지 않는 범주형 변수들도 포함하는 경우가 많지만 범주형 변수와 연속형 변수의 결합밀도함수(joint density function)를 설정하기 어려워 범주형 변수들이 정규분포를 따르는 것처럼 가정한 채 대체를 실시하는 경우가 흔히 발생한다. 본 논문에서는 이항반응 변수는 연속형 정규분포를 가진 잠재변수(latent variable)의 이분된(dichotomized) 형태라고 가정한 후 잠재변수의 정규분포를 이용하여 다중대체를 시행하고 대체된 값을 결절점(cutpoint)을 이용하여 다시 이항반응을 가진 변수로 변환하는 방법을 제안하였다. 또한, 이 방법은 순서형 변수(ordinal variable) 및 명목형 변수(nominal variables)로 확장되어 연속형 변수와 함께 다변량 정규분포 하에서 대체를 실시하는 것이 가능하다. 모의실험을 통하여 제안된 대체 방법의 성능이 연구되었는데 결측값을 무시한 채 분석을 실시하는 것 보다 정확한 평균 및 범주의 비율 추정이 가능한 것으로 나타났다.
When imputation is conducted under the parametric models, it is common to assume a multivariate normal distribution. Most real data include categorical variables as well as continuous variables. However, imputation models often assume normal distributions for categorical variables, since it is not easy to define a joint density function of continuous and categorical variables. In this study, we assume that a binary variable is a categorized form of a continuous latent variable that follows a normal distribution. Then, we can conduct multiple imputation using this continuous latent variable under the normal distribution assumption and obtain the binary variable from the latent variable using a cutpoint. Since this approach can be easily extended to handle ordinal variables and nominal variables, we can conduct multiple imputation of both continuous and categorical variables under the multivariate normal distribution assumption. A simulation study indicates that the suggested imputation model performs better than complete-case analysis.
1. 서론
2. 정규분포를 따르는 변수에 대한 다중대체법
3. 비정규성 분포를 따르는 변수에 대한 잠재변수를 사용한 다중대체법
4. 모의실험
5. 결론
참고문헌
(0)
(0)