베이지안 방법을 이용한 저부도포트폴리오 모형의 부도율 예측
Bayesian Inference of LDP Defaults Probabilities
- 한국자료분석학회
- Journal of The Korean Data Analysis Society (JKDAS)
- Vol.11 No.3
-
2009.061353 - 1362 (10 pages)
- 18
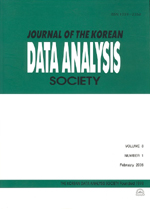
신용위험관리에서 해당 포트폴리오의 정확한 부도율 추정은 가장 중요한 이슈 가운데 하나이다. 그러나 신용도가 좋은 국가 및 금융기관 포트폴리오 또는 규모가 크거나 차주가 적은 특수금융 포트폴리오의 경우 부도 사건이 전혀 없거나 다년간에 걸쳐 매우 드물게 부도 사건이 나타나기 때문에 정확한 모형의 구축을 통한 부도율의 추정이 현실적으로 어렵다. 이와 같이 정상차주에 비해 부도차주가 매우 적거나, 부도차주가 없는 포트폴리오를 저부도포트폴리오(low default portfolio)라 한다. 본 연구에서는 저부도포트폴리오가 가지는 부도차주수의 한계를 극복하기 위해 베이지안 접근 방법에 기반한 부도예측 모형을 제안하고자 한다. 이를 위해 비정보사전정보(non- informative prior information)와 정보사전정보(informative prior information)을 혼합한 연속적사전정보를 사용하였는데, 연속적사전정보를 사용할 경우 부도차주수가 존재하지 않을 경우와 추정부도율의 서열화 문제를 동시에 해결할 수 있다.
One of aims in the credit risk management is to estimate a default probability significantly by maintaining the credit risk exposure within acceptable parameters. However, it is very difficult to construct an efficient credit risk model under the low default portfolios. Low default portfolios are those for which banks have little default history, so that average observed default rates might not be reliable estimators of default probabilities. In this paper, we suggest a default probability estimation method based on Bayesian inference in order to overcome the problem from the sparse default data. To do this, we compares with two types of prior information, where one is the non-informative prior and the other is the sequential prior as a informative prior. We can also confirm that the latter method is provide a reasonable result than the first one. Consequently, if we use the sequential prior information, we can solve such problems as deficiency of default data and unreasonable orders of estimates for probabilities.
1. 서론
2. 다중구간에서의 LDP에 대한 베이지안 추정방법
3. 사례연구
4. 결론
참고문헌
(0)
(0)