계층적 베이지안 방법을 이용한 용량반응연구에서의 기준용량 추정
Estimating a Benchmark Dose in Dose Response Studies via Bayesian Hierarchical Methods
- 한국자료분석학회
- Journal of The Korean Data Analysis Society (JKDAS)
- Vol.12 No.1
-
2010.02119 - 133 (15 pages)
- 13
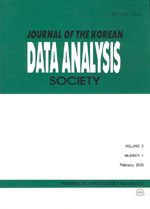
본 논문에서는 용량반응연구(dose response study)에서 많이 사용되고 있는 기준용량(benchmark dose)을 추정하기 위하여 계층적 베이지안 방법을 연구하였다. 이를 위하여 독성화학물질인 과염소산염(perchlorate)에 대한 두 가지 용량반응 사례 연구로부터 나오는 자료를 이용하여 계층적 베이지안 방법을 토대로 용량반응곡선을 추정하고 기준용량을 추정하고 계산하는 방법에 대하여 논의하였다. 과염소산염은 최근에 환경문제가 되고 있는 수질오염원의 하나로 알려져 있으며 과염소산염에 대한 과다 노출 시 갑상선기능에 영향을 주는 것으로 알려져 있다. 이러한 과염소산염에 대한 용량반응곡선을 계층적 베이지안 로지스틱 회귀모형을 이용하여 분석하였다. 구체적으로 계층적 사전분포를 바탕으로 사후추론을 실시하였고 이를 위하여 WinBUGS 프로그램으로 구현한 마르코프 연쇄 몬테칼로(Markov chain Monte Carlo, MCMC)방법을 이용하였다. 이러한 MCMC 방법을 통하여 추출된 사후표본을 이용하여 각각의 용량반응 자료에 대한 기준복용량을 계산하였다. 또한 두 용량반응자료의 추론을 결합하는 두 가지 방식을 제시하고 이를 통하여 이전에 추정된 기준용량보다 향상된 기준용량을 제시하였다.
In this paper, we develop a Bayesian hierarchical method for estimating a Benchmark dose(BMD) based on multiple health endpoints and informed by mechanistic knowledge of a biological process for a toxic chemical, perchlorate. Perchlorate is widespread water contaminant which acts as an iodine uptake inhibitor in the thyroid. A hierarchical approach is used to assign prior distribution of the unknow parameters. Posterior inference is performed via a Markov chain Monte Carlo(MCMC) method, implemented by WinBUGS, and MCMC outputs are used to determine Benchmark doses for each health endpoint. Specifically, we consider estimating BMDs for two dose-response studies with perchlorate, and discuss how to improve these BMD estimates by combining inferential procedures from both studies.
1. 서론
2. 자료 및 모형 설명
3. 계산 결과
4. 용량반응연구 결과의 결합
5. 요약 및 결론
참고문헌
(0)
(0)