네트워크 침입 탐지를 위한 의사결정나무 모형 적용에 대한 연구
A Study of Decision Tree in Detecting Intrusions
- 한국자료분석학회
- Journal of The Korean Data Analysis Society (JKDAS)
- Vol.12 No.2
-
2010.04983 - 996 (14 pages)
- 20
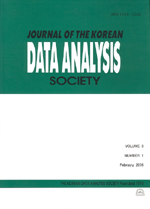
본 연구는 네트워크 침입을 탐지하기 위해 대표적인 데이터마이닝 방법론의 하나인 의사결정나무 모형을 사용하였다. 연구의 목적은 의사결정나무가 보안 분야에서 네트워크 공격을 얼마나잘 탐지하는가를 평가하고 적용가능성을 파악하는 것이다. 이를 위해 출력변수를 세 가지 형태로 구성하여 실험하였다; 공격유무, 정상적인 접속과 공격유형, 정상적인 접속과 22개의 세부공격내용. 492,298개의 자료로 의사결정나무를 학습하여 292,299개의 평가 자료로 예측력을 분석하였다. 실험결과 세 가지 형태의 출력변수에 대해 의사결정나무 모형의 정분류율에 대한 차이는유의하게 나타나지 않았고, 최소 97.71%의 정분류율을 보였다. 하지만 학습에 사용되지 않은 새로운 형태의 공격 유형을 기존에 학습된 의사결정나무 모형에 적용하였을 때 예측력은 급격하게떨어졌다. 따라서 의사결정나무 모형을 이용한 네트워크 침입탐지는 충분히 적용가능성을 보여주지만 새로운 침입방법이 생길 때마다 재학습하여 의사결정나무 모형을 갱신하는 것이 필요함을 시사한다.
This paper presents a study about the use of decision tree model to detect and classify intrusions. The aim of our research is to evaluate practicability of decision trees which classifies well the attacks and leads to the higher detection rate of each attack. This study focused on three classification types of output variable; a single class (normal or attack), Five attack categories or normal classes, and 22 types of attack or normal classes. A data set containing 492,298 records was trained in order to be tested on testing set containing 292,299 records. Our experiments show that the significant difference of detection accuracy do not exist among three types of output class and decision tree models have at least 97.71% of detection accuracy. But detection rate of trained decision tree was dramatically decreased for new types of attack which are not in the training data set. So decision tree model shows sufficient practicability in detecting intrusion but it must be rebuilded whenever new type of attack appear.
1. 서론
2. 침입탐지시스템과 주요 적용모델
3. 의사결정나무 모형 개요 및 평가 방안
4. 의사결정나무를 사용한 네트워크 침입탐지 실험
5. 결론
참고문헌
(0)
(0)