음이항 혼합회귀모형을 이용한 교통사고 자료분석 연구
Motor Vehicle Crash Count Data Analysis using Negative Binomial Mixture Regression Models
- 한국자료분석학회
- Journal of The Korean Data Analysis Society (JKDAS)
- Vol.12 No.3
-
2010.061485 - 1498 (14 pages)
- 26
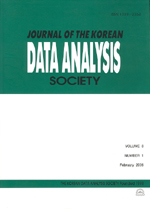
교통시설에 대한 안전성 연구에 있어서 차량사고 분석은 매우 중요하다. 특히 교통사고에 대한 통계적 분석을 통해 교통안전전문가들은 그 원인을 파악하고 궁극적으로 사고 발생건수와 그 정도를 줄일 수 있을 것이다. 최근 Park과 Lord(2009)는 교통사고 자료에 존재할 수 있는 이질성(heterogeneity)을 파악하기 위해 음이항 혼합회귀모형(negative binomial mixture regression model)을 이용하였다. 본 연구에서는 혼합회귀모형을 위해 모수적 조건부 질량함수(parametric conditional mass function)와 참 조건부 질량함수(true conditional mass function) 간의 L2 거리를 이용한 L2E 추정방법을 제안하였으며, Park과 Lord의 베이지안 방법 외에도 기존의 최대우도추정법과 L2E 방법을 바탕으로 2-성분 음이항 혼합회귀모형을 이용하여 캐나다 토론토의 교통사고 자료를 재분석하였다. 분석 결과, 계산의 용이성뿐만 아니라 공변량을 통한 해석의 적절성 측면에서 L2E 방법 역시 교통사고 발생건수에 대한 추정방법으로 우수한 것으로 나타났다.
It is important to analyze motor vehicle crashes in traffic safety studies. In particular, transportation safety analysts study the causes of traffic accidents and reduce the number of traffic accidents and severity of collisions via statistical analyses in a crash data. Recently, Park and Lord(2009) provided a Bayesian approach that could be used for capturing heterogeneity in a Toronto motor vehicle crash count data through the use of finite mixture regression models. In this study, we propose L2E estimation method for finite mixture regression models based on minimum integrated L2 distance between parametric conditional and true conditional mass functions. We also analyze Toronto Motor Vehicle Crashes of Park and Lord(2009) with the maximum likelihood and L2E estimations. We show that our L2E estimation for two-component mixture of negative binomial regression models provides a better overall fit in terms of capturing the heterogeneity in the count responses and providing the interpretability of component profiles via their respective covariates.
1. 서론
2. 이론적 배경
3. 자료 분석 및 비교 연구
4. 결론
참고문헌
(0)
(0)