앙상블 기법을 이용한 일반화가법모형 연구
An Ensemble Predictor for Generalized Additive Models
- 한국자료분석학회
- Journal of The Korean Data Analysis Society (JKDAS)
- Vol.17 No.5
-
2015.102403 - 2412 (10 pages)
- 30
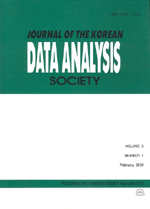
일반화가법모형(generalized additive models; GAM)은 선형 모형만이 아닌 평활함수를 사용하여 비선형 효과를 확인할 수 있는 장점이 있다. 많은 사회현상에서 비선형적 관계가 발견되므로 이를 설명하기 위한 방법론으로 널리 활용되고 있는 것이다. 한편, 많은 연구들에서 배깅, 랜덤포레스트 등의 앙상블(ensemble) 예측 기법이 단일 예측보다 더 좋은 성능을 가질 수 있음이 증명되었다. 특히 이러한 앙상블기법은 의사결정나무와 같이 예측값의 분산이 큰 분류자에 대해서만 주로 활용되고 있다. 최근 이항 반응변수에 대해 의사결정나무가 아닌 일반화가법모형을 단일 분류자로 하는 앙상블 분류자는 De Bock(2010), Kim, Lee(2013)에 의해 제안되었다. 본 연구에서는 일반화가법모형을 이용한 앙상블 예측 기법들의 특성을 살펴보고, 선형관계, 다항함수 관계, 삼각함수관계 등 비선형적 관계에 대한 모의실험을 통해 제안된 앙상블기법의 우수성을 확인하였다.
GAM (generalized additive model) has advantages which can find not only linear effects but nonlinear ones, since it can be widely used to real applications. Many studies have shown that ensemble methods such as bagging and random forests are better than the single predictor by making an accurate estimate. Especially, ensemble methods is used to improve performance of weak predictors such as decision trees. Recently, De Bock (2012) and Kim, Lee (2013) have proposed ensemble methods related to the base predictor derived from GAMs with the nominal and continuous response variable. In this study, we have tried to find the properties of the ensemble methods using simulation studies which include linear, polynomial, trigonometric function and Friedman (1991) data. We find that the ensemble methods are more efficient than previous one in the case of non-linear data.
1. 서론
2. 일반화가법모형과 앙상블 기법
3. 모의실험
4. 모의실험 결과
5. 결론
References
(0)
(0)