불균형 자료의 분류분석에서 샘플링 기법을 이용한 로지스틱 회귀분석
Logistic Regression with Sampling Techniques for the Classification of Imbalanced Data
- 한국자료분석학회
- Journal of The Korean Data Analysis Society (JKDAS)
- Vol.17 No.4
-
2015.081877 - 1888 (12 pages)
- 134
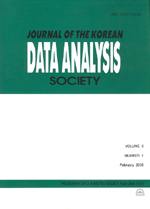
로지스틱 회귀분석(logistic regression)은 이항 범주형 자료의 분류분석에서 높은 분류정확도와 유연성을 바탕으로 다양한 분야에서 널리 활용되고 있다. 그러나 소수집단과 다수집단의 개체수가 현저하게 차이나는 불균형 자료(imbalanced data)의 분류분석에서 로지스틱 회귀분석은 다수집단에 편향된 분류함수를 추정하여 대부분의 자료를 다수집단으로 분류함으로써 소수집단의 분류 정확도가 현저히 감소하게 되는 제한사항이 있다. 따라서 로지스틱 회귀분석을 이용한 불균형 자료의 분류분석에서 소수집단의 분류 정확도를 높이기 위하여 본 논문에서는 다양한 샘플링 기법을 이용한 로지스틱 회귀분석 방법론에 대하여 연구하였다. 또한 설명변수(explanatory variable)가 고차원인 불균형 자료의 분류분석에서 잡음변수(noise variables)를 제거하고 중요한 설명변수들을 모형에 선택하기 위하여 라소 로지스틱 회귀분석(lasso logistic regression)에 샘플링 기법을 적용한 방법론에 대해서도 연구하였다. 본 논문에서는 모의실험과 실제자료의 분석을 통하여 분류정확도와 모형의 간결성 측면에서 제안한 방법론의 우수한 성능과 유용성을 확인하였다.
The logisitic regression is widely used in binary data classification areas with its flexibility and a high level of classification accuracy. However, when analyzing imbalanced data with different class sizes, the classification accuracy in minority class (sensitivity) may drop significantly because logistic regression classifiers is biased toward the majority class so that it classifies almost all observations to majority class. Therefore, we study logistic regression with various sampling technique to increase classification accuracy in minority class. Furthermore, we study lasso logistic regression in analyzing an imbalanced data not only to increase classification accuracy, but also to select important explanatory variables. In this study, we demonstrate the effectiveness of the proposed methods through simulation studies and a real data analysis in terms of classification accuracy and model selection.
1. 서론
2. 로지스틱 회귀분석과 라소 로지스틱 회귀분석
3. 불균형 자료의 분류분석을 위한 다양한 샘플링 기법
4. 모의 실험
5. 실제자료 분석
6. 결론
References
(0)
(0)