The Stock Index Prediction by the Time-Adjusted Classifier Ensemble
- 한국자료분석학회
- Journal of The Korean Data Analysis Society (JKDAS)
- Vol.17 No.4
-
2015.081747 - 1758 (12 pages)
- 2
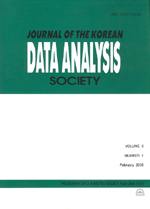
There are many time-related data in the world. The properties of the attributes change and they affect the target variable differently as time changes. For example, the oil price was the major indicator for the stock price several years ago. However, the gold price is more influential than the oil price to predict the stock price nowadays. Therefore, it is reasonable to give more weights for the recent instances when we predict the target variable from the past data set. Bagging and random forests use the bootstrapped training set to construct the algorithm. Here, we introduce the modified bootstrap method, the time-adjusted bootstrap which gives the recent instance more weight to be chosen instead of the equal weight for the conventional bootstrap. We experiment with financial data set to predict the movement of KOSPI (Korea composite stock price index). We compare them for 66 cases by the combination of the different data sets or the different degree of the weights for the robust conclusion. For 62 out of 66 cases, our method improves the accuracy over the original bootstrap and the average improvement rates are 3.2% and 2.0% for bagging and random forests respectively. Consequently, bagging with the modified bootstrap shows the better performance over the original bagging and random forests with the modified bootstrap also shows the better performance over the original random forests.
1. Introduction
2. Literature review
3. The proposed approach
4. Experimental study
5. Conclusion and Future Work
References
(0)
(0)