Weighted L₁ Multicategory Support Vector Machines for Unbalanced Cases
- 한국자료분석학회
- Journal of The Korean Data Analysis Society (JKDAS)
- Vol.17 No.3
-
2015.061175 - 1184 (10 pages)
- 2
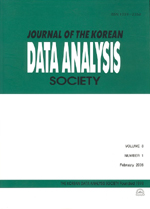
Classification has numerous applications in various real fields such as customer target marketing, medical disease diagnosis and so on. Among many classification methods the support vector machine (SVM) is very popular in real fields, because it has sound theoretical background and has a better performance than others. The SVM consists of the hinge loss function and the penalty function. The conventional SVM used the L₂ penalty, but it is well known that the SVM has drawbacks in variable selection especially when the predictor variables in the data has many noise variables. Furthermore, since standard SVM techniques typically treat all classes equally, it is not well suited to unbalanced cases, because the SVM minimizes the overall misclassification rate. Then the minor class can be neglected. We propose a weighted SVM method with the L₁ penalty function for unbalanced cases. The proposed method minimizes the within group misclassification rate. The method does not neglect the minor class which may have very important information on the data. Numerical simulation shows that the proposed method is effective for classification of the unbalanced data.
1. Introduction
2. Unbalanced SVM
3. Computation of Estimates
4. Numerical Experiment
5. Concluding Remarks
References
(0)
(0)