Moderately Clipped LASSO for the Sparse High-Dimensional Logistic Regression Models
- 한국자료분석학회
- Journal of The Korean Data Analysis Society (JKDAS)
- Vol.17 No.3
-
2015.061145 - 1154 (10 pages)
- 6
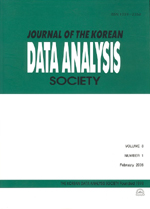
Many penalized estimation methods have been applied to the sparse high-dimensional logistic regression models for the classification tasks. Standard convex penalties such as the least absolute shrinkage and selection operator (LASSO) and ridge are known to have high prediction accuracy but tend to select too many irrelevant variables. On the other hand, standard concave penalties such as the smoothly clipped absolute deviation (SCAD) and minimax concave penalty (MCP) are known to successfully delete irrelevant variables but tend to be inferior to convex penalties in prediction accuracy. In this paper, we study a new penalty, the moderately clipped LASSO (MCL), in the sparse high-dimensional logistic regression models. The MCL is designed by combining the LASSO and MCP, and hence it is expected to preserve advantages of the LASSO and MCP simultaneously. We develop an efficient algorithm also. Numerical studies using simulated and real data analysis show that the MCL method successfully deletes irrelevant variables without losing prediction accuracy.
1. Introduction
2. Moderately clipped LASSO estimation
3. Numerical studies
4. Concluding remarks
References
(0)
(0)