은행과 저축은행을 분류하는 중요 재정 지표의 탐색
Key Financial Indexes Classifying Banks And Savings Banks
- 한국자료분석학회
- Journal of The Korean Data Analysis Society (JKDAS)
- Vol.15 No.2
-
2013.04719 - 730 (12 pages)
- 15
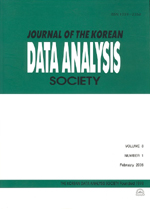
최근 우리나라 금융 기관 중 저축은행으로 인해 사회적으로 큰 문제가 발생되었다. 저축은행은 부동산 투자에 집중하였고 2008년 세계적으로 불어닥친 글로벌 경제 위기 속에서 부실을 키워 갔고 3차에 걸친 퇴출이 이루어졌다. 저축은행은 은행들과 구조적으로 다르고 사업행태도 다소 다른 면이 있다. 본 연구는 저축은행과 은행 간의 차이를 나타내는 재정 지표는 무엇이 있는가를 찾아내고자 하는데서 시작되었다. 금융기관들이 매우 많은 양의 지표들을 발생시키고 있는데 그 지표들(혹은 변수들)에 비하여 관측 대상(혹은 표본)의 수가 작은 고차원 데이터를 생산하고 있다. 실제로 본 연구의 경우, 증시에 상장된 5개 저축은행과 10개 은행이 만들어 내는 78개의 지표를 5년간 모은 데이터를 사용하였다. 따라서 컴퓨터상에 390×15의 행렬이 존재한다고 하겠다. 행렬의 크기도 실제로 매우 크지만 그로 인해 다중공선정의 문제가 발생하며 프로그램 수행 시 오류가 발생하여 분석이 수행되지 않는다. 그래서 저축은행과 은행을 판별해 낼 수 있는 중요한 지표를 찾기 위해 생물 정보학에서 마이크로어레이 데이터를 다루는 방법을 차용하여 분석을 수행하였다. 판별분석이 수행되기 전에 전처리과정으로 은행들을 구분해내는 유의미한 지표를 선택하여 선택된 지표들을 이용하여 판별분석을 수행하는 방법을 사용하였다. 그 결과 최종적으로 6개의 중요한 지표가 선택되었고 중요 지표들을 이용한 판별함수의 판별력이 100%에 이르렀다.
In Korea, there are two types of financial institutions: ‘banks’ and ‘savings banks’. Savings banks, once highly exposed to real estate project financing market, experienced rapid deterioration of their financial health during the global financial crisis in 2008 as real estate market went through subsequent recession. Since then, peoples are interested in which factors can classify banks and savings banks statistically. Such financial institutions are producing a huge amount of data where the number of variables is much more than the number of samples. In order to handle such a high dimensional data set, we propose to borrow some techniques from the field of bioinformatics. The data set we consider in this article has seventy-eight variables and fifteen samples for five years. Hence, we have a 390×15 matrix. This matrix is so huge to handle computationally and it may cause a multi-co linearity problem. We do first select significant variables out of seventy-eight variables by t-test, Šidák’s adjustment and significance analysis microarrays, and finally found sixteen very significant variables. Secondly cluster samples with significant variables, and finally derive discriminant functions with six important variables. Derived discriminant functions show 0% misclassification rate for analyzing a given example.
1. 서론
2. 분석방법
3. 실증분석
4. 결론
References
(0)
(0)