발생빈도와 상대적 사전 비율을 고려한 연관성 평가 모형의 개발
Utilization of Association Rule Thresholds Considering Frequency and Relatively Prior Rates
- 한국자료분석학회
- Journal of The Korean Data Analysis Society (JKDAS)
- Vol.15 No.2
-
2013.04709 - 718 (10 pages)
- 2
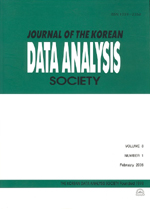
빅 데이터를 처리하는 것은 사용자 집단을 위해 허용 경과시간 내에 데이터를 관리하고 처리하는 범용 하드웨어 환경 및 소프트웨어 도구의 영역을 넘어선다. 이러한 환경에서 필요한 기술이 데이터마이닝 기법이다. 데이터마이닝 기법들 중에서 가장 활발하게 연구되고 있는 연관성 규칙은 항목들 간의 지지도, 신뢰도, 향상도 등의 연관성 규칙 평가 기준을 근거로 하여 항목들 간의 관련성을 탐색하는 데 활용되고 있다. 그런데 기존의 연관성 규칙 마이닝은 항목의 발생 유무만을 고려하여 규칙을 생성하여 왔으며, 이는 발생 빈도를 고려하지 않음으로써 정보 손실에 의한 오류를 범하거나 세밀하지 못한 해석을 할 수도 있다. 또한 연관성 규칙 생성과정의 첫 단계가 사용자가 지정한 최소 지지도의 조건을 만족하는 빈발항목집합을 생성하는 것인데, 희귀하게 발생되는 항목인 경우에는 빈발항목집합에 포함되지 않을 가능성이 매우 크다. 본 논문에서는 이 두 가지 문제를 동시에 해결하기 위해 항목의 발생빈도와 상대적 사전비율을 고려한 연관성 평가 모형을 개발하고자 한다.
Data mining is a powerful technology with great potential to help companies focus on the most important information in the massive database. The methods of data mining are decision tree, association rules, clustering, neural network and so on. Association rule mining is a popular and well researched method for discovering interesting relationships between itemsets in huge databases and has been applied in various fields. It is intended to identify strong rules discovered in large databases using different measures of interestingness. There are three primary quality measures for meaningful association rules; support, confidence, and lift. In this paper, we propose some association thresholds considering frequency and relatively prior occurrence rates for association rule exploration. The comparative studies with three kinds of supports and confidences are shown by numerical example. As a result, the higher frequency and relatively prior rates, the values of support and confidence considering frequency and relatively prior rates are greater than the existing supports and confidences.
1. 서론
2. 발생빈도와 상대적 사전 비율을 고려한 연관성 평가 기준
3. 예제를 통한 고찰
4. 결론
References
(0)
(0)