반응/미반응 자료의 과대표본 추출에 대한 연구
A Study for Oversampling under Rare Events Data
- 한국자료분석학회
- Journal of The Korean Data Analysis Society (JKDAS)
- Vol.15 No.4
-
2013.081883 - 1892 (10 pages)
- 12
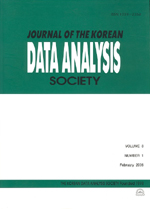
반응/미반응과 같은 이분형(binary) 목표변수를 갖는 모집단에서 모형개발을 위한 데이터마트를 형성할 때 반응/미반응 구성비는 구축된 모형의 성능에 영향을 준다. 따라서 목표변수의 특정 범주가 상대적으로 희소한 경우 모형 구축을 위하여 데이터마트를 형성할 때, 목표변수 각 범주 빈도의 수적 형평성을 맞추는 것이 바람직하다. 이를 과대표본추출(oversampling)이라고 한다. 본 연구는 이에 대한 실험적 연구로 과대표본추출의 구성비를 1:1에서 1:20까지 다양하게 구성하여 분류기준값(cut-off) 별로 최적모형을 찾아보았다. 특히 앙상블 기법인 부스팅(boosting) 중 아다부스트(AdaBoost)와 랜덤포레스트(random forests) 기법을 의사결정나무 및 회귀모형과 함께 비교하였다. 또한 교차타당성(cross-validation)을 통한 검증을 통하여 과대표본추출의 적절한 분류기준값과 구성비를 찾아보았다. 분류기준값 0.10에서 구성비가 1:20, 1:16 등 불균형이 심할 때는 로지스틱회귀분석이 좋은 결과를 주었으며 그 외의 구성비에서는 아다부스트가 가장 좋은 결과를 주었다. 분류기준값 0.20에서는 구성비의 불균형이 적을 때는 아다부스트가 좋은 결과를 주었으며 구성비의 불균형이 클 때는 의사결정나무가 좋은 결과를 주었다. 분류기준값 0.3에서 0.5까지에서는 로지스틱회귀분석과 랜덤포레스트가 좋은 결과를 주고 있으며 의사결정나무는 상대적으로 좋지 않은 결과를 보였다.
For binary classification problem, target ratio of data mart can affect model performance. When forming a data mart for model building, if certain categories of the target variable is relatively rare, it is desirable that good/bad ratio of target variable is balanced. This is called as oversampling. This study is experimental study for oversampling. Target ratio is changed from 1:1 to 1:20. We found optimal model under various target ratio. Especially, boosting and random forrest are compared with traditional classification method decision tree and logistic regression. Proper target ratio and cut-off value are found by cross-validation. Logistic regression model gave better result when target/non-target ratio is 1:20 and 1:16 under cut-off value 0.10. AdaBoost gave the best result for other target ratio cases. Under the cut-off value 0.20, AdaBoost gave better result for target/non-target ratio is balanced decision tree gave better result for unbalanced target/non-target ratio. Under the cut-off value 0.3 to 0.5, logistic regression and random forest gave better result while decision tree gave worse result.
1. 서론
2. 앙상블 기법
3. 과대표본추출
4. 모형개발을 위한 자료준비
5. 과대표본추출의 구성비별 모형개발 및 평가
6. 결론 및 토의
References
(0)
(0)