협력적 필터링 추천시스템에서 유의성 가중치의 영향에 관한 연구
A Study on the Influence of Significance Weight in Collaborative Filtering Recommender Systems
- 한국자료분석학회
- Journal of The Korean Data Analysis Society (JKDAS)
- Vol.15 No.6
-
2013.123141 - 3148 (8 pages)
- 7
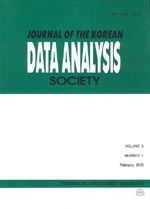
본 논문의 목적은 전자상거래에서 상품을 고객에게 추천하는 추천시스템의 알고리즘 중 상품에 대한 고객의 명시적 선호도 평가치를 이용하여 특정 상품에 대한 고객의 선호도를 평가하는 협력적 필터링 알고리즘의 선호도 예측 성능을 향상시키는데 있다. 협력적 필터링 알고리즘에서 고객 간 선호도의 유사 정도를 나타내는 유사도 가중치는 일반적으로 피어슨 상관계수가 이용되지만 상관계수는 공통으로 평가한 선호도 평가치 개수가 작을 경우 과대평가되는 경향이 있다. 기존연구에서 공통으로 평가된 평가치 개수의 영향을 보정하기 위해 사용된 유의성 가중치는 공통 평가치 개수가 적을 경우 영향을 줄이도록 적용하였지만 본 연구에서는 기존 연구의 적용방법과 달리 상관계수에 공통평가치의 개수를 직접 가중시키는 방법으로 유의성 가중치를 적용하였다. 결과에서 기존에 제시된 유의성 가중치를 변형한 가중치를 적용함으로써 예측의 정확도를 향상시킬 수 있었다.
The purpose of this research is dedicated to improve prediction accuracy of collaborative filtering algorithms which estimate the active user’s preference level to specific item with only using explicit ratings of other users’ and active user’s ratings. Pearson’s correlation coefficient as the similarity weight which measures the degree of similarity between active user and each user’s preference to items is generally used on collaborative filtering algorithm. But if the numbers of co-rated ratings are small, the calculated similarity weight usually tends to over estimated. In precedent research, the significance weight is used to adjust the influence of the number of co-rated ratings and the aim of significance weight is that to lower the influence of correlation coefficient in case of the number of co-rated items are small. This research apply improved significance weight which multiplies similarity weight to soften the influence of the number of co-rated items. This proposed significance weight is different from that was used in early study. In the results, we improve the prediction accuracy of collaborative filtering algorithm by applying proposed significance weight.
1. 서론
2. 관련연구
3. 연구방법
4. 실험결과
5. 결론
References
(0)
(0)