결측이 있는 영과잉 가산형 자료에 대한 포아송 모형의 모수 추정
Parameter Estimation of Zero-Inflated Poisson Model for Incomplete Count Data
- 한국자료분석학회
- Journal of The Korean Data Analysis Society (JKDAS)
- Vol.16 No.4
-
2014.081849 - 1857 (9 pages)
- 13
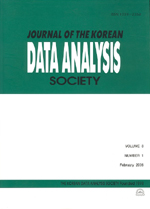
가산형(count) 자료에 대한 분석은 포아송 분포를 가정하여 실시하는데 이 때 포아송 분포에서 기대하는 것보다 0의 값을 가진 관찰치가 많은 경우 영과잉 포아송 분포(zero-inflated Poisson distribution)를 가정하여 분석을 실시할 수 있다. Lambert(1992)는 영과잉 포아송 모형에 대하여 EM 알고리즘을 통한 모수 추정 방법을 제안하였다. 가산형 반응 변수에 결측이 발생하는 경우 결측자료 메커니즘이 MCAR이 아니라면 결측이 발생한 자료를 제외한 채 완전한 자료 만에 근거한 분석을 실시하면 모수의 추정에 편향이 발생할 수 있다. 본 연구에서는 영과잉 가산형 자료에 결측이 발생하는 경우 적용할 수 있는 모수 추정 방법으로서 MCEM 알고리즘을 통한 모수 추정 방법을 제안한다. 모의실험을 통해 영과잉 포아송 모형에서 결측이 발생하는 경우 결측자료 메커니즘이 MAR이라면 결측된 자료를 제외한 채 완전하게 응답된 자료 만에 근거한 분석은 편향이 발생할 수 있음을 보이고 본 연구에서 제안한 MCEM 알고리즘을 통해 모수를 추정한다면 추정치에 편향이 덜 발생한다는 것을 보였다. 제안된 방법을 실제 자료에 적용하는 예제로서 낚은 물고기 숫자에 대한 영과잉 포아송 모형의 적합 결과를 설명하였다.
Count data are analyzed under the Poisson distribution assumption. When Data contain more zeros than expected under the Poisson distribution, it is possible to analyze the data using the zero-inflated Poisson distribution. Lambert (1992) proposed the EM (expectation maximization) algorithm to estimate parameters of the zero-inflated Poisson model. When the count response variable includes missing values, complete-case analysis may produce biased parameter estimates if the missing data mechanism is not MCAR (missing completely at random). We propose the MCEM (Monte Carlo expectation maximization) algorithm to estimate parameters of the zero-inflated Poisson model when the count response variable is sometimes missing. Simulation is conducted to show that complete-case analysis can result in biased parameter estimates and the propose MCEM algorithm provide less biased parameter estimates under the MAR (missing at random) mechanism. Fishing data are analyzed to explain how to apply the proposed method to real data.
1. 서론
2. 영과잉 포아송 모형의 모수 추정
3. 모의실험
4. 낚시 자료에 대한 적용
5. 토의 및 결론
References
(0)
(0)