허들모형에 대한 베이지안 추론
Bayesian Inference for Hurdle Models
- 한국자료분석학회
- Journal of The Korean Data Analysis Society (JKDAS)
- Vol.16 No.4
-
2014.081837 - 1847 (11 pages)
- 9
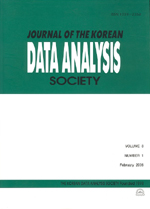
영과잉 개수형 자료는 개수형 자료에서 영(0)의 값이 가정된 분포에 비하여 과다하게 나타나는 형태의 자료를 의미한다. 이러한 자료에 대한 분석으로 본 연구에서는 포아송 및 음이항 허들모형을 고려하고, 이들 모형에 대하여 마코프체인 몬테카를로(Markov chain Monte Carlo; MCMC) 기법을 이용한 베이지안 추론방법을 제안하고자 한다. 음이항 허들모형은 개수형 자료에서 흔히 발생하는 과대산포를 허용해주는 모형이다. 두 모형에 대한 회귀계수를 추정하고자 마코프체인 몬테카를로 알고리즘을 사용하였다. 본 연구에서는 메트로폴리스 해스팅스 알고리즘(metropolis hastings algorithm)과 깁스 알고리즘(Gibbs algorithm)을 결합한 방법을 이용하였다. 아울러 본 연구에서 제안한 두 모형에 Gurmu, Trivedi(1996)에 의하여 분석된 휴양항해 자료를 적용하여 두 모형의 효율성을 DIC(deviance information criterion)를 이용하여 비교하였다. 자료분석 결과, 음이항 허들모형의 DIC값이 포아송 허들모형의 DIC값 보다 작게 나타나 음이항 허들모형이 포아송 허들모형보다 잘 적합 되었음을 알 수 있었다.
In practice, count data often show a higher incidence of zero counts than expected for the assumed distribution. Such data are called the zero inflated count data. To analyse zero inflated count data, we consider the Poisson and negative binomial hurdle model, and propose the Bayesian inference using Markov chain Monte Carlo (MCMC) methods. Especially, the negative binomial hurdle model allows the overdispersion of dependent variable. In these models, we propose the MCMC algorithm compromising the metropolis hastings algorithm and Gibbs sampler to estimate regression parameters. In order to compare the efficiencies of the proposed models, we apply the proposed models to a well known the recreational trips data analysed by Gurmu, Trivedi (1996). The deviance information criterion (DIC) was used to compare the goodness of fit of each model. From the result of real data analysis, the negative binomial hurdle model shows the better performance than the Poisson hurdle model, since the DIC of negative binomial hurdle model is smaller than that of Poisson hurdle model.
1. 서론
2. 허들모형
3. 사전분포와 사후분포
4. DIC를 이용한 베이지안 모형비교
5. 자료의 분석
6. 결론
References
(0)
(0)