A Sparse Ridge Estimation for the Sparse Logistic Regression Model
- 한국자료분석학회
- Journal of The Korean Data Analysis Society (JKDAS)
- Vol.16 No.4
-
2014.081715 - 1725 (11 pages)
- 7
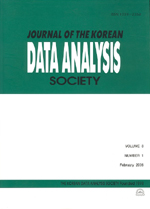
In this paper, we study a new penalized maximum likelihood estimator, the sparse ridge, for the sparse logistic regression model. Here, the sparsity of the logistic model implies that the model includes some irrelevant predictive variables. Hence, by deleting the irrelevant predictive variables from the model, we want to identify a correct sub-model, enhancing both prediction accuracy and model interpretability. For the problem, the sparse ridge gives a simultaneous process of selecting relevant predictive variables and estimating included parameters. First, the sparse ridge achieves a sparsity as usual penalized maximum likelihood estimators such as the least absolute selection and shrinkage operator and smoothly clipped absolute deviation penalized estimators. Hence we can use the sparse ridge for variable selection for the sparse logistic regression model. Further, the sparse ridge shares a common shrinkage effect in parameter estimation with the ridge. Hence the sparse ridge is expected to perform well under the multi-collinearity, especially. Numerical studies via simulated and real data analysis show that the sparse ridge can be a nice alternative to the well-known penalized maximum likelihood estimators.
1. Introduction
2. Sparse ridge estimation
3. Numerical Studies
4. Concluding Remarks
References
(0)
(0)