범주형 자료에 대한 군집분석 비교연구
Comparison of Clustering Methods for Categorical Data
- 한국자료분석학회
- Journal of The Korean Data Analysis Society (JKDAS)
- Vol.16 No.5
-
2014.102439 - 2445 (7 pages)
- 107
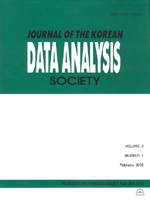
고객만족도 조사연구에서 개별 리커트 척도 사용은 매우 일반적이다. 이러한 리커트 척도에 의해 얻어진 데이터에 대한 군집분석은 주의를 기울일 필요가 있다. 보통 많이 적용하고 있는 일반적 군집분석은 연속적 데이터 즉 수치형 척도에 의해 얻어진 데이터에 적합한 경우가 대부분이다. 반면 리커트 척도와 같은 이산형 혹은 범주형 데이터에 대한 군집분석 방법은 최근 연구가 많이 이루어지고 있는 실정이며 본 연구에서는 Hamming 거리를 이용한 군집기법, 이단계(two-step) 군집분석 그리고 k-means 방법과 비교를 하고 이중 가장 성능이 우수한 방법으로 실제 사례에 적용해본다. 비교는 Fisher의 붓꽃자료를 이산형으로 변형한 데이터로 모의실험을 하였고, 이때 가장 정분류율이 높은 이단계 군집방법을 이용하여 D대학 2013년 학생 만족도 조사로 얻어진 범주형 자료에 대한 군집분석을 적용하여 보았다. 군집분석을 통하여 충성도가 높은 학생 군과 낮은 학생 군을 찾고 두 군간의 특징으로 학점을 고려하였으나 통계적 차이는 나타나지 않았다.
In customers satisfaction survey, using individual (not summated) Likert-type items as measurement tools is common. When the data is obtained by Likert type scales, the cluster analysis must be performed carefully. In general, many clustering techniques were developed for the continuous data. However, a large amount of categorical data is coming from various research areas. Recently, new techniques have been developed for analysis of such a kind of the data. In this paper, we compare some clustering methods, that is, clustering based on Hamming distance, two-step clustering and k-means clustering using modified Fisher’s Iris data. As a result of small simulation, two-step method is the best of all in correction rates. Thus, we applied the two step clustering to the student satisfaction survey data of D university. Then we found the two clusters, one is a satisfied group and the other is a unsatisfied group.
1. 서론
2. 범주형 측도에 대한 군집방법 소개
3. 모의실험
4. 사례분석
5. 결론
References
(0)
(0)