결합기법을 이용한 요양보험 등급판정 모형 연구
Predictions of Functional Conditions in Long-Term Care Insurances Using Ensemble Predictor
- 한국자료분석학회
- Journal of The Korean Data Analysis Society (JKDAS)
- Vol.16 No.5
-
2014.102409 - 2416 (8 pages)
- 26
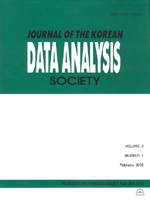
요양보험 대상자에 대한 요양인정점수의 산출 및 등급판정 도구는 노인장기요양보험제도의 신뢰성에 있어 매우 중요한 요소 중 하나이다. 현재 사용하고 있는 요양인정점수 산출 도구는 데이터마이닝의 방법론인 의사결정분석(decision tree)의 결과를 이용하여 개발된 것이다. 최근의 연구에서는 일화가법모형(generalized additive model)을 통해 의사결정나무에서 발생하였던 등급판정 자료의 역전현상 및 비선형관계를 설명할 수 있는 도구로 활용될 수 있음을 보였다. 본 연구에서는 일반화가법모형에서 나아가 결합기법(ensemble)을 이용한 모형을 판정도구로 고려하였다. 결합기법은 배깅(bagging), 랜덤포레스트(random forest) 등과 같이 단일 예측보다 더 좋은 성능을 보이는 것으로 알려져 있다. 따라서 등급판정도구에 일반화가법모형을 기본 분류자로 하는 결합기법을 고려하였으며 실제 자료 분석결과 등급판정에서 우수한 결과를 보임을 확인하였다.
The prediction model is a very important factor to support the long-term insurance system. The present prediction model is decision tree as one of datamining techniques. In recent studies, the generalized additive model (GAM) could be the alternatives of decision tree model as assessment models in long-term care insurance because GAMs may explain the non-linearity of data. In this study, we extend the GAMs to ensemble models for prediction of functional conditions. It is known that ensemble models, such as bagging, random forests have better prediction performances than single predictor’s. We consider a ensemble model using GAMs as base predictor to assess the level of functional conditions. As a results, we find that the ensemble model has good performances of the long-term insurance data.
1. 서론
2. 데이터
3. 일반화가법모형을 이용한 결합기법
4. 요양등급 판정 자료의 적합
5. 결론
References
(0)
(0)