Geostatistical Data Analysis with Outlier Detection
- 한국자료분석학회
- Journal of The Korean Data Analysis Society (JKDAS)
- Vol.16 No.5
-
2014.102285 - 2297 (13 pages)
- 7
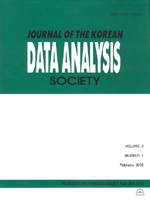
Recently, researchers of the various fields where the spatial analysis is needed have demonstrated more interest in spatial statistics. Spatial data consist of observations measured at specific locations or within specific regions. In this study, we focus on geostatistical data among three spatial data types that are dealt with in spatial statistics. Geostatistical data are analyzed in three stages: estimation of the variogram, model fit for the estimated variogram, and prediction of the value at specified locations using the fitted variogram model. Because variogram modeling is significantly affected by outliers, methods to detect and remove outliers from data sets are critical for proper variogram modeling. In this paper, we propose a procedure to detect outliers for spatial data analysis. Here, to detect outliers, we use the sample influence function (SIF) for the Akaike information criterion (AIC) and the maximum likelihood function. By applying our approach to artificial data and an empirical example with rainfall data in Chugoku, Japan, we are able to demonstrate the performance and usefulness of our proposed method.
1. Introduction
2. Spatial Prediction
3. Outlier Detection
4. Simulation Study
5. Case Study
6. Conclusions
References
(0)
(0)