두 구성비 데이터 집단의 차이 추론을 위한 ALR 변환과 ILR 변환의 비교
Comparison of ALR and ILR Transformations for Inference of the Difference between Two Groups of Compositional Data
- 한국자료분석학회
- Journal of The Korean Data Analysis Society (JKDAS)
- Vol.16 No.6
-
2014.123023 - 3036 (14 pages)
- 14
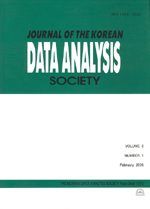
이 연구는 구성비 데이터로 이루어진 두 집단을 비교하고자 한다. 두 집단을 비교하는 것은 통계적 추론의 전형적인 문제들 중의 하나이다. 구성비 데이터와 같은 다변량 데이터가 수반될 때 흔히 다변량 분석이 시도된다. 구성비 데이터는 모든 성분이 양수이고 그들의 합이 1로 제한되는 벡터들로 구성된다. 구성비 데이터의 표본공간은 단위 심플렉스이다. 이런 특수성은 구성비 데이터에 유클리드 공간상의 데이터를 위해 고안된 표준적인 다변량분석 방법들을 적용하기 어렵게 만든다. ALR(additive logratio) 변환과 ILR(isometric lograito) 변환은 심플렉스 공간과 유클리드 공간 간에 1:1 대응을 제공한다. 우리는 정규분포와 로그정규분포를 토대로 생성된 두 구성비 데이터 집단들의 차이에 대한 추론에서 이 두 가지 변환의 성능을 비교하였다. 그 결과, 이 두 종류의 데이터 원천 모두에 대해 ALR 변환이 더 좋은 성능을 보여 주었다.
This study tries to compare two groups of compositional data. Comparing two groups is one of the problems typical of statistical inference. When multivariate data such as compositional data is included, multivariate analyses are often tried. The compositional data is composed of vectors such that all components of them are strictly positive and their sum is constrained to 1. The sample space of the compositional data is the unit simplex. Its peculiarities prevent us from applying the standard multivariate statistical techniques designed for Euclidean spaces. ALR (additive logratio) transformation and ILR (isometric lograito) transformation provides the one-to-one correspondences between the simplex space and the Euclidean space. In this thesis, ALR and ILR transformations for inferring the difference between two groups of the compositional data (each derived from normal distribution and lognormal distribution) are compared. As a result, when compositional data derived from normal distribution or lognormal distribution, ALR transformation is more appropriate way to infer the difference between two groups of the compositional data.
1. 서론
2. 구성비 데이터의 변환과 정규성
3. 격자 검정에 의한 두 구성비 데이터 집단의 비교
4. 수치 예에 의한 ALR 변환과 ILR 변환의 성능비교
5. 결론
References
(0)
(0)