표본 크기의 추정에서 붓스트랩 직접법을 이용한 로버스트 방법
Robust Method for Sample Size Estimation Using Bootstrap Aggregation
- 한국자료분석학회
- Journal of The Korean Data Analysis Society (JKDAS)
- Vol.17 No.2
-
2015.04729 - 737 (9 pages)
- 13
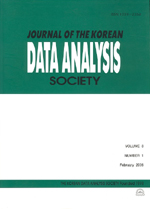
임상 연구와 표본 조사에서 추출할 표본의 크기를 결정하는 작업은 통계적 연구의 첫 단계로서 실험이나 연구의 품질을 결정하는 매우 중요한 단계이다. 많은 경우에 대규모 실험이나 연구를 진행하기 전에 규모가 작은 표본을 추출하여 모집단의 특성에 대한 정보를 얻고 이를 기반으로 목표 정도를 만족할 수 있는 표본의 크기를 결정한다. 소규모 표본을 추출하여 모집단의 정보를 알아보는 시험조사(pilot study)에서는 표본의 크기의 제약으로 인하여 모집단에 대한 추정량의 분산이 매우 큰 경우가 많다. 본조사를 위하여 시험조사를 빈번히 실시하는 통계작성기관이나 연구기관은 작은 표본으로부터 얻어진 표본 크기의 추정값이 큰 변동성 가지기 때문에 시간과 비용을 낭비하거나 목표한 결과를 얻지 못하게 되는 경우가 많다. 본 논문에서는 중앙값을 이용한 붓스트랩 집적법을 이용하여 작은 표본에 기초한 표본 크기의 추정량의 변동을 줄이는 방법을 제안하고 그 효과를 모의실험으로 제시하고자 한다.
Sample size calculation plays an important role in clinical trials and survey research for detecting a meaningful difference at a pre-specified level of significance. In order to determine the minimum sample size required to guarantee the intended power, one often needs to know the values of a number of parameters such as mean and standard deviation of population. In practice, however, the values of these parameters are often unknown. The estimates of these unknown parameters based on data from a small pilot study are often used without any consideration about the associated large sampling variation or impact of outlier. In this article, we show that this common practice for sample size determination may lead to a seriously biased and unstable sample size. As a simple solution, we propose a bootstrap aggregation approach using median, which is able to select the required minimum sample size with a substantially improved stability. Monte Carlo simulations demonstrate that performance of the proposed method has substantial improvement over the traditional method.
1. 서론
2. 붓스트랩 집적법을 이용한 표본 크기의 결정
3. 모의실험
4. 결론
References
(0)
(0)